# Lay Perceptions of Crowd-Scientific Findings **The Risks of Variability and Lack of Consensus** [Shilaan Alzahawi](http://shilaan.rbind.io) & [Benoît Monin](https://www.gsb.stanford.edu/faculty-research/faculty/benoit-monin) .pull-left[ 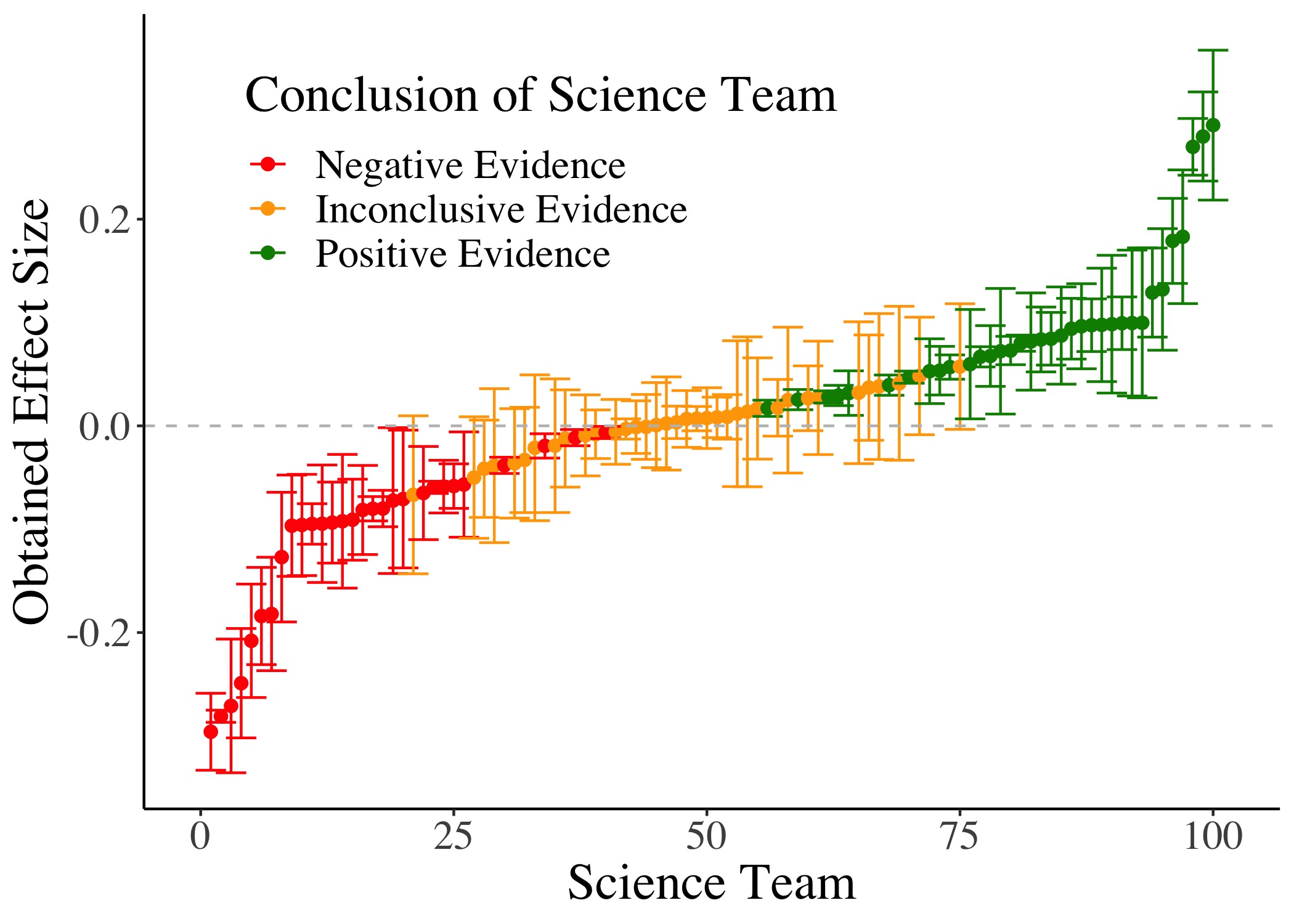 ] .pull-right[ `\(~~\)` [<svg viewBox="0 0 512 512" style="position:relative;display:inline-block;top:.1em;fill:#035AA6;height:2em;" xmlns="http://www.w3.org/2000/svg"> <path d="M459.37 151.716c.325 4.548.325 9.097.325 13.645 0 138.72-105.583 298.558-298.558 298.558-59.452 0-114.68-17.219-161.137-47.106 8.447.974 16.568 1.299 25.34 1.299 49.055 0 94.213-16.568 130.274-44.832-46.132-.975-84.792-31.188-98.112-72.772 6.498.974 12.995 1.624 19.818 1.624 9.421 0 18.843-1.3 27.614-3.573-48.081-9.747-84.143-51.98-84.143-102.985v-1.299c13.969 7.797 30.214 12.67 47.431 13.319-28.264-18.843-46.781-51.005-46.781-87.391 0-19.492 5.197-37.36 14.294-52.954 51.655 63.675 129.3 105.258 216.365 109.807-1.624-7.797-2.599-15.918-2.599-24.04 0-57.828 46.782-104.934 104.934-104.934 30.213 0 57.502 12.67 76.67 33.137 23.715-4.548 46.456-13.32 66.599-25.34-7.798 24.366-24.366 44.833-46.132 57.827 21.117-2.273 41.584-8.122 60.426-16.243-14.292 20.791-32.161 39.308-52.628 54.253z"></path></svg> `\(~~\)` twitter.com/shilaan01](https://twitter.com/shilaan01) [<svg viewBox="0 0 496 512" style="position:relative;display:inline-block;top:.1em;fill:#035AA6;height:2em;" xmlns="http://www.w3.org/2000/svg"> <path d="M336.5 160C322 70.7 287.8 8 248 8s-74 62.7-88.5 152h177zM152 256c0 22.2 1.2 43.5 3.3 64h185.3c2.1-20.5 3.3-41.8 3.3-64s-1.2-43.5-3.3-64H155.3c-2.1 20.5-3.3 41.8-3.3 64zm324.7-96c-28.6-67.9-86.5-120.4-158-141.6 24.4 33.8 41.2 84.7 50 141.6h108zM177.2 18.4C105.8 39.6 47.8 92.1 19.3 160h108c8.7-56.9 25.5-107.8 49.9-141.6zM487.4 192H372.7c2.1 21 3.3 42.5 3.3 64s-1.2 43-3.3 64h114.6c5.5-20.5 8.6-41.8 8.6-64s-3.1-43.5-8.5-64zM120 256c0-21.5 1.2-43 3.3-64H8.6C3.2 212.5 0 233.8 0 256s3.2 43.5 8.6 64h114.6c-2-21-3.2-42.5-3.2-64zm39.5 96c14.5 89.3 48.7 152 88.5 152s74-62.7 88.5-152h-177zm159.3 141.6c71.4-21.2 129.4-73.7 158-141.6h-108c-8.8 56.9-25.6 107.8-50 141.6zM19.3 352c28.6 67.9 86.5 120.4 158 141.6-24.4-33.8-41.2-84.7-50-141.6h-108z"></path></svg> `\(~~\)` shilaan.rbind.io](https://shilaan.rbind.io) [<svg viewBox="0 0 496 512" style="position:relative;display:inline-block;top:.1em;fill:#035AA6;height:2em;" xmlns="http://www.w3.org/2000/svg"> <path d="M165.9 397.4c0 2-2.3 3.6-5.2 3.6-3.3.3-5.6-1.3-5.6-3.6 0-2 2.3-3.6 5.2-3.6 3-.3 5.6 1.3 5.6 3.6zm-31.1-4.5c-.7 2 1.3 4.3 4.3 4.9 2.6 1 5.6 0 6.2-2s-1.3-4.3-4.3-5.2c-2.6-.7-5.5.3-6.2 2.3zm44.2-1.7c-2.9.7-4.9 2.6-4.6 4.9.3 2 2.9 3.3 5.9 2.6 2.9-.7 4.9-2.6 4.6-4.6-.3-1.9-3-3.2-5.9-2.9zM244.8 8C106.1 8 0 113.3 0 252c0 110.9 69.8 205.8 169.5 239.2 12.8 2.3 17.3-5.6 17.3-12.1 0-6.2-.3-40.4-.3-61.4 0 0-70 15-84.7-29.8 0 0-11.4-29.1-27.8-36.6 0 0-22.9-15.7 1.6-15.4 0 0 24.9 2 38.6 25.8 21.9 38.6 58.6 27.5 72.9 20.9 2.3-16 8.8-27.1 16-33.7-55.9-6.2-112.3-14.3-112.3-110.5 0-27.5 7.6-41.3 23.6-58.9-2.6-6.5-11.1-33.3 2.6-67.9 20.9-6.5 69 27 69 27 20-5.6 41.5-8.5 62.8-8.5s42.8 2.9 62.8 8.5c0 0 48.1-33.6 69-27 13.7 34.7 5.2 61.4 2.6 67.9 16 17.7 25.8 31.5 25.8 58.9 0 96.5-58.9 104.2-114.8 110.5 9.2 7.9 17 22.9 17 46.4 0 33.7-.3 75.4-.3 83.6 0 6.5 4.6 14.4 17.3 12.1C428.2 457.8 496 362.9 496 252 496 113.3 383.5 8 244.8 8zM97.2 352.9c-1.3 1-1 3.3.7 5.2 1.6 1.6 3.9 2.3 5.2 1 1.3-1 1-3.3-.7-5.2-1.6-1.6-3.9-2.3-5.2-1zm-10.8-8.1c-.7 1.3.3 2.9 2.3 3.9 1.6 1 3.6.7 4.3-.7.7-1.3-.3-2.9-2.3-3.9-2-.6-3.6-.3-4.3.7zm32.4 35.6c-1.6 1.3-1 4.3 1.3 6.2 2.3 2.3 5.2 2.6 6.5 1 1.3-1.3.7-4.3-1.3-6.2-2.2-2.3-5.2-2.6-6.5-1zm-11.4-14.7c-1.6 1-1.6 3.6 0 5.9 1.6 2.3 4.3 3.3 5.6 2.3 1.6-1.3 1.6-3.9 0-6.2-1.4-2.3-4-3.3-5.6-2z"></path></svg> `\(~~\)` github.com/shilaan](https://github.com/shilaan) [<svg viewBox="0 0 512 512" style="position:relative;display:inline-block;top:.1em;fill:#035AA6;height:2em;" xmlns="http://www.w3.org/2000/svg"> <path d="M440 6.5L24 246.4c-34.4 19.9-31.1 70.8 5.7 85.9L144 379.6V464c0 46.4 59.2 65.5 86.6 28.6l43.8-59.1 111.9 46.2c5.9 2.4 12.1 3.6 18.3 3.6 8.2 0 16.3-2.1 23.6-6.2 12.8-7.2 21.6-20 23.9-34.5l59.4-387.2c6.1-40.1-36.9-68.8-71.5-48.9zM192 464v-64.6l36.6 15.1L192 464zm212.6-28.7l-153.8-63.5L391 169.5c10.7-15.5-9.5-33.5-23.7-21.2L155.8 332.6 48 288 464 48l-59.4 387.3z"></path></svg> `\(~~\)` shilaan@stanford.edu](mailto:shilaan@stanford.edu) ] ??? Hi, thank you for being here. Today I'll present some recent findings on crowdsourcing data analysis that I've been working on in collaboration with my co-author Professor Benoit Monin. --- # Outline ??? First, I'll share a brief open science statement and links to all the available materials. Then, I'll define crowd science. -- <svg viewBox="0 0 576 512" style="position:relative;display:inline-block;top:.1em;fill:#035AA6;height:2em;" xmlns="http://www.w3.org/2000/svg"> <path d="M423.5 0C339.5.3 272 69.5 272 153.5V224H48c-26.5 0-48 21.5-48 48v192c0 26.5 21.5 48 48 48h352c26.5 0 48-21.5 48-48V272c0-26.5-21.5-48-48-48h-48v-71.1c0-39.6 31.7-72.5 71.3-72.9 40-.4 72.7 32.1 72.7 72v80c0 13.3 10.7 24 24 24h32c13.3 0 24-10.7 24-24v-80C576 68 507.5-.3 423.5 0z"></path></svg> `\(~~\)` [**Open Science Statement**](#openscience) -- <svg viewBox="0 0 640 512" style="position:relative;display:inline-block;top:.1em;fill:#035AA6;height:2em;" xmlns="http://www.w3.org/2000/svg"> <path d="M96 224c35.3 0 64-28.7 64-64s-28.7-64-64-64-64 28.7-64 64 28.7 64 64 64zm448 0c35.3 0 64-28.7 64-64s-28.7-64-64-64-64 28.7-64 64 28.7 64 64 64zm32 32h-64c-17.6 0-33.5 7.1-45.1 18.6 40.3 22.1 68.9 62 75.1 109.4h66c17.7 0 32-14.3 32-32v-32c0-35.3-28.7-64-64-64zm-256 0c61.9 0 112-50.1 112-112S381.9 32 320 32 208 82.1 208 144s50.1 112 112 112zm76.8 32h-8.3c-20.8 10-43.9 16-68.5 16s-47.6-6-68.5-16h-8.3C179.6 288 128 339.6 128 403.2V432c0 26.5 21.5 48 48 48h288c26.5 0 48-21.5 48-48v-28.8c0-63.6-51.6-115.2-115.2-115.2zm-223.7-13.4C161.5 263.1 145.6 256 128 256H64c-35.3 0-64 28.7-64 64v32c0 17.7 14.3 32 32 32h65.9c6.3-47.4 34.9-87.3 75.2-109.4z"></path></svg> `\(~\)` [**Crowdsourcing Science**](#crowdscience) ??? Then, I'll define crowd science and crowdsourced data analysis and introduce our question of interest, which is "does crowdsourcing data analysis improve lay perceptions of scientific findings?". -- <svg viewBox="0 0 512 512" style="position:relative;display:inline-block;top:.1em;fill:#035AA6;height:2em;" xmlns="http://www.w3.org/2000/svg"> <path d="M396.8 352h22.4c6.4 0 12.8-6.4 12.8-12.8V108.8c0-6.4-6.4-12.8-12.8-12.8h-22.4c-6.4 0-12.8 6.4-12.8 12.8v230.4c0 6.4 6.4 12.8 12.8 12.8zm-192 0h22.4c6.4 0 12.8-6.4 12.8-12.8V140.8c0-6.4-6.4-12.8-12.8-12.8h-22.4c-6.4 0-12.8 6.4-12.8 12.8v198.4c0 6.4 6.4 12.8 12.8 12.8zm96 0h22.4c6.4 0 12.8-6.4 12.8-12.8V204.8c0-6.4-6.4-12.8-12.8-12.8h-22.4c-6.4 0-12.8 6.4-12.8 12.8v134.4c0 6.4 6.4 12.8 12.8 12.8zM496 400H48V80c0-8.84-7.16-16-16-16H16C7.16 64 0 71.16 0 80v336c0 17.67 14.33 32 32 32h464c8.84 0 16-7.16 16-16v-16c0-8.84-7.16-16-16-16zm-387.2-48h22.4c6.4 0 12.8-6.4 12.8-12.8v-70.4c0-6.4-6.4-12.8-12.8-12.8h-22.4c-6.4 0-12.8 6.4-12.8 12.8v70.4c0 6.4 6.4 12.8 12.8 12.8z"></path></svg> `\(~~~\)` [**Crowdsourcing Data Analysis**](#crowddata) -- <svg viewBox="0 0 512 512" style="position:relative;display:inline-block;top:.1em;fill:#035AA6;height:2em;" xmlns="http://www.w3.org/2000/svg"> <path d="M256 8C119.043 8 8 119.083 8 256c0 136.997 111.043 248 248 248s248-111.003 248-248C504 119.083 392.957 8 256 8zm0 448c-110.532 0-200-89.431-200-200 0-110.495 89.472-200 200-200 110.491 0 200 89.471 200 200 0 110.53-89.431 200-200 200zm107.244-255.2c0 67.052-72.421 68.084-72.421 92.863V300c0 6.627-5.373 12-12 12h-45.647c-6.627 0-12-5.373-12-12v-8.659c0-35.745 27.1-50.034 47.579-61.516 17.561-9.845 28.324-16.541 28.324-29.579 0-17.246-21.999-28.693-39.784-28.693-23.189 0-33.894 10.977-48.942 29.969-4.057 5.12-11.46 6.071-16.666 2.124l-27.824-21.098c-5.107-3.872-6.251-11.066-2.644-16.363C184.846 131.491 214.94 112 261.794 112c49.071 0 101.45 38.304 101.45 88.8zM298 368c0 23.159-18.841 42-42 42s-42-18.841-42-42 18.841-42 42-42 42 18.841 42 42z"></path></svg> `\(~~~\)` [**Lay Perceptions of Crowd-Scientific Findings**](#lay) -- <svg viewBox="0 0 512 512" style="position:relative;display:inline-block;top:.1em;fill:#035AA6;height:2em;" xmlns="http://www.w3.org/2000/svg"> <path d="M501.1 395.7L384 278.6c-23.1-23.1-57.6-27.6-85.4-13.9L192 158.1V96L64 0 0 64l96 128h62.1l106.6 106.6c-13.6 27.8-9.2 62.3 13.9 85.4l117.1 117.1c14.6 14.6 38.2 14.6 52.7 0l52.7-52.7c14.5-14.6 14.5-38.2 0-52.7zM331.7 225c28.3 0 54.9 11 74.9 31l19.4 19.4c15.8-6.9 30.8-16.5 43.8-29.5 37.1-37.1 49.7-89.3 37.9-136.7-2.2-9-13.5-12.1-20.1-5.5l-74.4 74.4-67.9-11.3L334 98.9l74.4-74.4c6.6-6.6 3.4-17.9-5.7-20.2-47.4-11.7-99.6.9-136.6 37.9-28.5 28.5-41.9 66.1-41.2 103.6l82.1 82.1c8.1-1.9 16.5-2.9 24.7-2.9zm-103.9 82l-56.7-56.7L18.7 402.8c-25 25-25 65.5 0 90.5s65.5 25 90.5 0l123.6-123.6c-7.6-19.9-9.9-41.6-5-62.7zM64 472c-13.2 0-24-10.8-24-24 0-13.3 10.7-24 24-24s24 10.7 24 24c0 13.2-10.7 24-24 24z"></path></svg> `\(~~~\)` [**Method**](#method) -- <svg viewBox="0 0 512 512" style="position:relative;display:inline-block;top:.1em;fill:#035AA6;height:2em;" xmlns="http://www.w3.org/2000/svg"> <path d="M496 384H64V80c0-8.84-7.16-16-16-16H16C7.16 64 0 71.16 0 80v336c0 17.67 14.33 32 32 32h464c8.84 0 16-7.16 16-16v-32c0-8.84-7.16-16-16-16zM464 96H345.94c-21.38 0-32.09 25.85-16.97 40.97l32.4 32.4L288 242.75l-73.37-73.37c-12.5-12.5-32.76-12.5-45.25 0l-68.69 68.69c-6.25 6.25-6.25 16.38 0 22.63l22.62 22.62c6.25 6.25 16.38 6.25 22.63 0L192 237.25l73.37 73.37c12.5 12.5 32.76 12.5 45.25 0l96-96 32.4 32.4c15.12 15.12 40.97 4.41 40.97-16.97V112c.01-8.84-7.15-16-15.99-16z"></path></svg> `\(~~~\)` [**Results**](#results) ??? Afterwards we'll briefly talk about the methods and results of an experiment, and hopefully we'll have some time at the end for some discussion. -- <svg viewBox="0 0 512 512" style="position:relative;display:inline-block;top:.1em;fill:#035AA6;height:2em;" xmlns="http://www.w3.org/2000/svg"> <path d="M256 32C114.6 32 0 125.1 0 240c0 47.6 19.9 91.2 52.9 126.3C38 405.7 7 439.1 6.5 439.5c-6.6 7-8.4 17.2-4.6 26S14.4 480 24 480c61.5 0 110-25.7 139.1-46.3C192 442.8 223.2 448 256 448c141.4 0 256-93.1 256-208S397.4 32 256 32zm0 368c-26.7 0-53.1-4.1-78.4-12.1l-22.7-7.2-19.5 13.8c-14.3 10.1-33.9 21.4-57.5 29 7.3-12.1 14.4-25.7 19.9-40.2l10.6-28.1-20.6-21.8C69.7 314.1 48 282.2 48 240c0-88.2 93.3-160 208-160s208 71.8 208 160-93.3 160-208 160z"></path></svg> `\(~~~\)` [**Discussion**](#discussion) ??? If you have any questions, thoughts, comments, please feel free to raise your hand or unmute yourself and jump in. We have quite some time today for this session, so I'd be happy to make this as interactive as you'd like it to be. --- class: inverse, middle, center name: openscience # Open Science Statement --- background-image: url("https://github.com/allisonhorst/stats-illustrations/raw/master/rstats-artwork/reproducibility_court.png") background-position: 90% 50% background-size: 50% # Open Science Statement -- [<svg viewBox="0 0 512 512" style="position:relative;display:inline-block;top:.1em;fill:#035AA6;height:2em;" xmlns="http://www.w3.org/2000/svg"> <g groupmode="layer" id="layer6" label="icon"> <path id="path246" style="fill-opacity:1;fill-rule:nonzero;stroke:none;stroke-width:0.0352777" d="m 255.96701,7.9999992 c -14.42056,0 -28.9134,3.1667198 -39.84197,9.5105508 L 69.402174,102.20596 c -21.954611,12.67476 -39.899208,43.75438 -39.899208,69.08441 v 169.41924 c 0,25.33653 17.944597,56.42568 39.899208,69.0939 L 216.12504,494.51788 C 227.05361,500.84232 241.54645,504 255.96701,504 c 14.48556,0 28.97013,-3.15768 39.89917,-9.48212 l 146.7224,-84.71437 c 21.92213,-12.66822 39.90845,-43.75737 39.90845,-69.0939 V 171.29037 c 0,-25.33003 -17.98632,-56.40965 -39.90845,-69.08441 L 295.86618,17.51055 C 284.93714,11.166719 270.45257,7.9999992 255.96701,7.9999992 Z M 355.1558,152.37339 c 4.22726,0 8.45019,1.60138 11.65118,4.81215 l 17.84669,17.82761 c 6.402,6.42793 6.402,16.93826 0,23.3597 L 228.22149,354.82393 c -6.46654,6.41499 -16.93871,6.41499 -23.34074,0 l -68.94211,-68.91386 c -6.40202,-6.41497 -5.044,-18.2773 0,-23.34073 l 17.85586,-17.82761 c 6.40202,-6.42793 16.9387,-6.42793 23.34073,0 l 27.74552,27.72655 c 6.40203,6.415 16.8742,6.415 23.34074,0 L 343.45702,157.18554 c 3.23307,-3.21072 7.47102,-4.81215 11.69878,-4.81215 z"></path> </g></svg> `\(~~~\)` Preregistration](https://osf.io/rpu98) -- [<svg viewBox="0 0 512 512" style="position:relative;display:inline-block;top:.1em;fill:#035AA6;height:2em;" xmlns="http://www.w3.org/2000/svg"> <g groupmode="layer" id="layer6" label="icon"> <path id="path86" style="fill-opacity:1;fill-rule:nonzero;stroke:none;stroke-width:0.0352777" d="m 255.99051,7.9999992 c -14.45957,0 -28.91558,3.1667158 -39.88958,9.5105468 L 69.397238,102.20596 C 47.455739,114.88072 29.50769,145.96034 29.50769,171.29036 v 169.41925 c 0,25.33653 17.948049,56.42568 39.889548,69.0939 l 146.703692,84.71437 c 10.974,6.32444 25.4365,9.48212 39.88958,9.48212 14.46596,0 28.92849,-3.15768 39.88953,-9.48212 l 146.72261,-84.71437 c 21.9352,-12.66822 39.88966,-43.75737 39.88966,-69.0939 V 171.29036 c 0,-25.33002 -17.95446,-56.40964 -39.88966,-69.0844 L 295.88952,17.510546 c -10.974,-6.343831 -25.43305,-9.5105468 -39.89901,-9.5105468 z m -58.05822,145.7374608 58.06766,22.86711 58.09612,-22.86711 100.07901,40.79892 -58.06755,22.85762 V 317.49208 L 255.99995,358.27204 155.90196,317.49208 V 217.394 L 97.815363,194.53638 Z M 155.90196,217.394 255.99995,258.16451 356.10753,217.394 255.99995,176.60457 Z"></path> </g></svg> `\(~~~\)` Open materials](https://osf.io/6vze3) -- [<svg viewBox="0 0 512 512" style="position:relative;display:inline-block;top:.1em;fill:#035AA6;height:2em;" xmlns="http://www.w3.org/2000/svg"> <g groupmode="layer" id="layer6" label="icon"> <path id="path90" style="fill-opacity:1;fill-rule:nonzero;stroke:none;stroke-width:0.0352777" d="M 255.99349,8.0446377 C 223.80996,6.8710755 198.88846,29.106812 171.90108,43.078975 135.19601,64.83668 97.398702,84.909644 61.424592,107.79531 c -23.529448,19.12486 -34.340913,49.89944 -31.869027,79.61823 0.136467,52.69231 -0.273926,105.39637 0.206898,158.08127 3.046554,31.90013 25.166618,58.97257 53.630746,72.31691 45.693451,26.22554 91.119371,52.93874 136.981251,78.85735 29.15402,13.29373 63.66608,7.7032 89.45702,-10.28262 45.55933,-26.46403 91.40188,-52.46098 136.78286,-79.21917 26.10444,-18.58601 38.49713,-51.27731 35.81722,-82.60095 -0.13644,-52.69193 0.27402,-105.39561 -0.20674,-158.08014 C 479.17554,134.58854 457.05921,107.51038 428.59249,94.170602 382.89744,67.950466 337.46312,41.255709 291.60404,15.334368 280.50454,10.177688 268.1747,8.0789301 255.99349,8.0446377 Z M 227.29819,140.73479 c 19.13338,0 38.26677,0 57.40015,0 0,76.83994 0,153.67988 0,230.51981 -19.13338,0 -38.26677,0 -57.40015,0 0,-76.83993 0,-153.67987 0,-230.51981 z m 72.23096,50.17436 c 19.12396,0 38.2479,0 57.37188,0 0,60.11515 0,120.2303 0,180.34545 -19.12398,0 -38.24792,0 -57.37188,0 0,-60.11515 0,-120.2303 0,-180.34545 z m -144.45251,94.07924 c 19.13025,0 38.26049,0 57.39074,0 0,28.7554 0,57.51081 0,86.26621 -19.13025,0 -38.26049,0 -57.39074,0 0,-28.7554 0,-57.51081 0,-86.26621 z"></path> </g></svg> `\(~~~\)` Open data](https://osf.io/b527v/) -- [<svg viewBox="0 0 581 512" style="position:relative;display:inline-block;top:.1em;fill:#035AA6;height:2em;" xmlns="http://www.w3.org/2000/svg"> <path d="M581 226.6C581 119.1 450.9 32 290.5 32S0 119.1 0 226.6C0 322.4 103.3 402 239.4 418.1V480h99.1v-61.5c24.3-2.7 47.6-7.4 69.4-13.9L448 480h112l-67.4-113.7c54.5-35.4 88.4-84.9 88.4-139.7zm-466.8 14.5c0-73.5 98.9-133 220.8-133s211.9 40.7 211.9 133c0 50.1-26.5 85-70.3 106.4-2.4-1.6-4.7-2.9-6.4-3.7-10.2-5.2-27.8-10.5-27.8-10.5s86.6-6.4 86.6-92.7-90.6-87.9-90.6-87.9h-199V361c-74.1-21.5-125.2-67.1-125.2-119.9zm225.1 38.3v-55.6c57.8 0 87.8-6.8 87.8 27.3 0 36.5-38.2 28.3-87.8 28.3zm-.9 72.5H365c10.8 0 18.9 11.7 24 19.2-16.1 1.9-33 2.8-50.6 2.9v-22.1z"></path></svg> `\(~~\)` Reproducible manuscript](https://github.com/shilaan/Many-Analysts/tree/main/manuscript) -- [<svg viewBox="0 0 512 512" style="position:relative;display:inline-block;top:.1em;fill:#035AA6;height:2em;" xmlns="http://www.w3.org/2000/svg"> <g label="icon" id="layer6" groupmode="layer"> <path id="path2" d="m 255.9997,7.9999987 c -34.36057,0 -62.21509,27.8545563 -62.21509,62.2151643 0,20.303056 9.87066,38.160947 24.91769,49.517247 0.18814,-20.457899 16.79601,-36.993393 37.29685,-36.993393 20.50082,0 37.11091,16.535494 37.29909,36.993393 15.04533,-11.3563 24.9177,-29.212506 24.9177,-49.517247 C 318.21272,35.854555 290.35915,7.9999987 255.99915,7.9999987 Z M 293.29654,392.2676 c -0.18814,20.4601 -16.79601,36.99338 -37.29684,36.99338 -20.50082,0 -37.10922,-16.53551 -37.29684,-36.99338 -15.04759,11.35627 -24.91769,29.21246 -24.91769,49.51722 0,34.36059 27.85453,62.21518 62.2151,62.21518 34.36056,0 62.21508,-27.85459 62.21508,-62.21518 0,-20.30306 -9.87066,-38.16095 -24.91767,-49.51722 z M 441.78489,193.78484 c -20.30301,0 -38.16309,9.87068 -49.51717,24.91769 20.45786,0.18819 36.99333,16.79605 36.99333,37.29689 0,20.50085 -16.53547,37.11096 -36.9911,37.29916 11.35634,15.04533 29.21249,24.91769 49.51721,24.91769 C 476.14549,318.21327 504,290.35948 504,255.99942 504,221.6394 476.14549,193.78425 441.78489,193.78425 Z M 82.738898,255.99997 c 0,-20.50139 16.535509,-37.11096 36.993392,-37.29689 -11.35632,-15.04756 -29.214164,-24.91769 -49.517197,-24.91769 -34.36057,0 -62.2150945,27.85455 -62.2150945,62.21517 0,34.3606 27.8545245,62.21516 62.2150945,62.21516 20.303033,0 38.160877,-9.87068 49.517197,-24.91773 -20.457883,-0.18818 -36.993391,-16.796 -36.993391,-37.29688 z M 431.3627,80.636814 c -24.29549,-24.295544 -63.68834,-24.295544 -87.9844,0 -14.35704,14.357057 -20.00298,33.963346 -17.39331,52.633806 -0.0824,0.0809 -0.18198,0.13437 -0.26434,0.21491 -14.578,14.57799 -14.578,38.21689 0,52.79488 14.57797,14.57799 38.21681,14.57799 52.79484,0 0.0824,-0.0824 0.13455,-0.18198 0.21732,-0.26434 18.66819,2.60796 38.27445,-3.03799 52.63151,-17.39336 24.29378,-24.29778 24.29378,-63.68837 -0.003,-87.986153 z M 186.2806,378.51178 c 14.57798,-14.57799 14.57798,-38.21461 0,-52.79319 -14.57798,-14.57853 -38.21683,-14.57798 -52.79481,0 -0.0825,0.0824 -0.13448,0.18199 -0.21476,0.26215 -18.67046,-2.60795 -38.276723,3.03572 -52.63376,17.39505 -24.297753,24.29552 -24.297753,63.6884 0,87.98449 24.29551,24.29552 63.68833,24.29552 87.98439,0 14.35702,-14.35703 20.00297,-33.96333 17.39333,-52.63386 0.0848,-0.0786 0.18364,-0.13228 0.26672,-0.21505 z m 0,-245.02583 c -0.0826,-0.0824 -0.18198,-0.13436 -0.26445,-0.21494 2.60795,-18.66823 -3.038,-38.27452 -17.39332,-52.633811 -24.29777,-24.295544 -63.68832,-24.295544 -87.984405,0 -24.297747,24.297781 -24.297747,63.688381 0,87.986151 14.357042,14.35706 33.963315,20.00301 52.631515,17.39336 0.0808,0.0824 0.13447,0.18199 0.21475,0.26434 14.57799,14.57799 38.21684,14.57799 52.79482,0 14.57797,-14.57802 14.57797,-38.21689 0,-52.79488 z m 245.0821,209.89048 c -14.35703,-14.35703 -33.96329,-20.00301 -52.63378,-17.39505 -0.0809,-0.0824 -0.13228,-0.18199 -0.21506,-0.26215 -14.57797,-14.57799 -38.21685,-14.57799 -52.79482,0 -14.57797,14.57799 -14.57797,38.21461 0,52.79316 0.0827,0.0828 0.18198,0.13455 0.26434,0.21505 -2.60796,18.67053 3.03802,38.27683 17.39334,52.63386 24.29552,24.29552 63.68834,24.29552 87.98439,0 24.29775,-24.29552 24.29775,-63.68841 0.003,-87.98451 z" style="stroke-width:0.07717"></path> </g></svg> `\(~~~\)` osf.io/vedb4](https://osf.io/vedb4) -- [<svg viewBox="0 0 496 512" style="position:relative;display:inline-block;top:.1em;fill:#035AA6;height:2em;" xmlns="http://www.w3.org/2000/svg"> <path d="M165.9 397.4c0 2-2.3 3.6-5.2 3.6-3.3.3-5.6-1.3-5.6-3.6 0-2 2.3-3.6 5.2-3.6 3-.3 5.6 1.3 5.6 3.6zm-31.1-4.5c-.7 2 1.3 4.3 4.3 4.9 2.6 1 5.6 0 6.2-2s-1.3-4.3-4.3-5.2c-2.6-.7-5.5.3-6.2 2.3zm44.2-1.7c-2.9.7-4.9 2.6-4.6 4.9.3 2 2.9 3.3 5.9 2.6 2.9-.7 4.9-2.6 4.6-4.6-.3-1.9-3-3.2-5.9-2.9zM244.8 8C106.1 8 0 113.3 0 252c0 110.9 69.8 205.8 169.5 239.2 12.8 2.3 17.3-5.6 17.3-12.1 0-6.2-.3-40.4-.3-61.4 0 0-70 15-84.7-29.8 0 0-11.4-29.1-27.8-36.6 0 0-22.9-15.7 1.6-15.4 0 0 24.9 2 38.6 25.8 21.9 38.6 58.6 27.5 72.9 20.9 2.3-16 8.8-27.1 16-33.7-55.9-6.2-112.3-14.3-112.3-110.5 0-27.5 7.6-41.3 23.6-58.9-2.6-6.5-11.1-33.3 2.6-67.9 20.9-6.5 69 27 69 27 20-5.6 41.5-8.5 62.8-8.5s42.8 2.9 62.8 8.5c0 0 48.1-33.6 69-27 13.7 34.7 5.2 61.4 2.6 67.9 16 17.7 25.8 31.5 25.8 58.9 0 96.5-58.9 104.2-114.8 110.5 9.2 7.9 17 22.9 17 46.4 0 33.7-.3 75.4-.3 83.6 0 6.5 4.6 14.4 17.3 12.1C428.2 457.8 496 362.9 496 252 496 113.3 383.5 8 244.8 8zM97.2 352.9c-1.3 1-1 3.3.7 5.2 1.6 1.6 3.9 2.3 5.2 1 1.3-1 1-3.3-.7-5.2-1.6-1.6-3.9-2.3-5.2-1zm-10.8-8.1c-.7 1.3.3 2.9 2.3 3.9 1.6 1 3.6.7 4.3-.7.7-1.3-.3-2.9-2.3-3.9-2-.6-3.6-.3-4.3.7zm32.4 35.6c-1.6 1.3-1 4.3 1.3 6.2 2.3 2.3 5.2 2.6 6.5 1 1.3-1.3.7-4.3-1.3-6.2-2.2-2.3-5.2-2.6-6.5-1zm-11.4-14.7c-1.6 1-1.6 3.6 0 5.9 1.6 2.3 4.3 3.3 5.6 2.3 1.6-1.3 1.6-3.9 0-6.2-1.4-2.3-4-3.3-5.6-2z"></path></svg> `\(~~~\)` github.com/shilaan/many-analysts](https://github.com/shilaan/many-analysts) -- [<svg viewBox="0 0 640 512" style="position:relative;display:inline-block;top:.1em;fill:#035AA6;height:1.7em;" xmlns="http://www.w3.org/2000/svg"> <path d="M592 0H48A48 48 0 0 0 0 48v320a48 48 0 0 0 48 48h240v32H112a16 16 0 0 0-16 16v32a16 16 0 0 0 16 16h416a16 16 0 0 0 16-16v-32a16 16 0 0 0-16-16H352v-32h240a48 48 0 0 0 48-48V48a48 48 0 0 0-48-48zm-16 352H64V64h512z"></path></svg> `\(~~\)` Slides at bit.ly/many-analysts](https://bit.ly/many-analysts) .right[ Artwork by [@allison_horst](https://github.com/allisonhorst/stats-illustrations) ] ??? ??? In terms of the materials, these are all available online, including a preregistration, the survey, data and code, and a fully reproducible manuscript. You can find all these materials on the OSF and on Github, and you can also find these slides online if you want to have them in front of you at bit.ly/many-analysts. --- class: inverse, center, middle name: crowdscience # Crowdsourcing Science --- .center[ # Crowd Science ] -- ### The organization of scientific research in open and collaborative projects -- From highly **inclusive** (sourcing citizens) to highly **selective** (sourcing specialists) -- Used at different **stages** of the research process ??? You may have already heard of crowdfunding, which uses the crowd for funding, but you can also use a crowd of individuals for generating research ideas, designing studies, collecting data, and analyzing data, which is what I'll be talking about today. -- .pull-left[ .pull-left[ <svg viewBox="0 0 512 512" style="position:relative;display:inline-block;top:.1em;fill:#035AA6;height:1em;" xmlns="http://www.w3.org/2000/svg"> <path d="M0 405.3V448c0 35.3 86 64 192 64s192-28.7 192-64v-42.7C342.7 434.4 267.2 448 192 448S41.3 434.4 0 405.3zM320 128c106 0 192-28.7 192-64S426 0 320 0 128 28.7 128 64s86 64 192 64zM0 300.4V352c0 35.3 86 64 192 64s192-28.7 192-64v-51.6c-41.3 34-116.9 51.6-192 51.6S41.3 334.4 0 300.4zm416 11c57.3-11.1 96-31.7 96-55.4v-42.7c-23.2 16.4-57.3 27.6-96 34.5v63.6zM192 160C86 160 0 195.8 0 240s86 80 192 80 192-35.8 192-80-86-80-192-80zm219.3 56.3c60-10.8 100.7-32 100.7-56.3v-42.7c-35.5 25.1-96.5 38.6-160.7 41.8 29.5 14.3 51.2 33.5 60 57.2z"></path></svg> `\(~~~\)` **Funding** <svg viewBox="0 0 352 512" style="position:relative;display:inline-block;top:.1em;fill:#035AA6;height:1em;" xmlns="http://www.w3.org/2000/svg"> <path d="M176 80c-52.94 0-96 43.06-96 96 0 8.84 7.16 16 16 16s16-7.16 16-16c0-35.3 28.72-64 64-64 8.84 0 16-7.16 16-16s-7.16-16-16-16zM96.06 459.17c0 3.15.93 6.22 2.68 8.84l24.51 36.84c2.97 4.46 7.97 7.14 13.32 7.14h78.85c5.36 0 10.36-2.68 13.32-7.14l24.51-36.84c1.74-2.62 2.67-5.7 2.68-8.84l.05-43.18H96.02l.04 43.18zM176 0C73.72 0 0 82.97 0 176c0 44.37 16.45 84.85 43.56 115.78 16.64 18.99 42.74 58.8 52.42 92.16v.06h48v-.12c-.01-4.77-.72-9.51-2.15-14.07-5.59-17.81-22.82-64.77-62.17-109.67-20.54-23.43-31.52-53.15-31.61-84.14-.2-73.64 59.67-128 127.95-128 70.58 0 128 57.42 128 128 0 30.97-11.24 60.85-31.65 84.14-39.11 44.61-56.42 91.47-62.1 109.46a47.507 47.507 0 0 0-2.22 14.3v.1h48v-.05c9.68-33.37 35.78-73.18 52.42-92.16C335.55 260.85 352 220.37 352 176 352 78.8 273.2 0 176 0z"></path></svg> `\(~~~~\)` **Ideation** <svg viewBox="0 0 640 512" style="position:relative;display:inline-block;top:.1em;fill:#035AA6;height:1em;" xmlns="http://www.w3.org/2000/svg"> <path d="M384 320H256c-17.67 0-32 14.33-32 32v128c0 17.67 14.33 32 32 32h128c17.67 0 32-14.33 32-32V352c0-17.67-14.33-32-32-32zM192 32c0-17.67-14.33-32-32-32H32C14.33 0 0 14.33 0 32v128c0 17.67 14.33 32 32 32h95.72l73.16 128.04C211.98 300.98 232.4 288 256 288h.28L192 175.51V128h224V64H192V32zM608 0H480c-17.67 0-32 14.33-32 32v128c0 17.67 14.33 32 32 32h128c17.67 0 32-14.33 32-32V32c0-17.67-14.33-32-32-32z"></path></svg> `\(~~\)` **Study design** <svg viewBox="0 0 576 512" style="position:relative;display:inline-block;top:.1em;fill:#035AA6;height:1em;" xmlns="http://www.w3.org/2000/svg"> <path d="M527.9 224H480v-48c0-26.5-21.5-48-48-48H272l-64-64H48C21.5 64 0 85.5 0 112v288c0 26.5 21.5 48 48 48h400c16.5 0 31.9-8.5 40.7-22.6l79.9-128c20-31.9-3-73.4-40.7-73.4zM48 118c0-3.3 2.7-6 6-6h134.1l64 64H426c3.3 0 6 2.7 6 6v42H152c-16.8 0-32.4 8.8-41.1 23.2L48 351.4zm400 282H72l77.2-128H528z"></path></svg> `\(~~\)` **Data collection** ] .pull-right[ <svg viewBox="0 0 640 512" style="position:relative;display:inline-block;top:.1em;fill:#035AA6;height:1em;" xmlns="http://www.w3.org/2000/svg"> <path d="M255.03 261.65c6.25 6.25 16.38 6.25 22.63 0l11.31-11.31c6.25-6.25 6.25-16.38 0-22.63L253.25 192l35.71-35.72c6.25-6.25 6.25-16.38 0-22.63l-11.31-11.31c-6.25-6.25-16.38-6.25-22.63 0l-58.34 58.34c-6.25 6.25-6.25 16.38 0 22.63l58.35 58.34zm96.01-11.3l11.31 11.31c6.25 6.25 16.38 6.25 22.63 0l58.34-58.34c6.25-6.25 6.25-16.38 0-22.63l-58.34-58.34c-6.25-6.25-16.38-6.25-22.63 0l-11.31 11.31c-6.25 6.25-6.25 16.38 0 22.63L386.75 192l-35.71 35.72c-6.25 6.25-6.25 16.38 0 22.63zM624 416H381.54c-.74 19.81-14.71 32-32.74 32H288c-18.69 0-33.02-17.47-32.77-32H16c-8.8 0-16 7.2-16 16v16c0 35.2 28.8 64 64 64h512c35.2 0 64-28.8 64-64v-16c0-8.8-7.2-16-16-16zM576 48c0-26.4-21.6-48-48-48H112C85.6 0 64 21.6 64 48v336h512V48zm-64 272H128V64h384v256z"></path></svg> `\(~~\)` **Data analysis** <svg viewBox="0 0 512 512" style="position:relative;display:inline-block;top:.1em;fill:#035AA6;height:1em;" xmlns="http://www.w3.org/2000/svg"> <path d="M512 0C460.22 3.56 96.44 38.2 71.01 287.61c-3.09 26.66-4.84 53.44-5.99 80.24l178.87-178.69c6.25-6.25 16.4-6.25 22.65 0s6.25 16.38 0 22.63L7.04 471.03c-9.38 9.37-9.38 24.57 0 33.94 9.38 9.37 24.59 9.37 33.98 0l57.13-57.07c42.09-.14 84.15-2.53 125.96-7.36 53.48-5.44 97.02-26.47 132.58-56.54H255.74l146.79-48.88c11.25-14.89 21.37-30.71 30.45-47.12h-81.14l106.54-53.21C500.29 132.86 510.19 26.26 512 0z"></path></svg> `\(~~~\)` **Writing** <svg viewBox="0 0 384 512" style="position:relative;display:inline-block;top:.1em;fill:#035AA6;height:1em;" xmlns="http://www.w3.org/2000/svg"> <path d="M369.9 97.9L286 14C277 5 264.8-.1 252.1-.1H48C21.5 0 0 21.5 0 48v416c0 26.5 21.5 48 48 48h288c26.5 0 48-21.5 48-48V131.9c0-12.7-5.1-25-14.1-34zM332.1 128H256V51.9l76.1 76.1zM48 464V48h160v104c0 13.3 10.7 24 24 24h104v288H48zm250.2-143.7c-12.2-12-47-8.7-64.4-6.5-17.2-10.5-28.7-25-36.8-46.3 3.9-16.1 10.1-40.6 5.4-56-4.2-26.2-37.8-23.6-42.6-5.9-4.4 16.1-.4 38.5 7 67.1-10 23.9-24.9 56-35.4 74.4-20 10.3-47 26.2-51 46.2-3.3 15.8 26 55.2 76.1-31.2 22.4-7.4 46.8-16.5 68.4-20.1 18.9 10.2 41 17 55.8 17 25.5 0 28-28.2 17.5-38.7zm-198.1 77.8c5.1-13.7 24.5-29.5 30.4-35-19 30.3-30.4 35.7-30.4 35zm81.6-190.6c7.4 0 6.7 32.1 1.8 40.8-4.4-13.9-4.3-40.8-1.8-40.8zm-24.4 136.6c9.7-16.9 18-37 24.7-54.7 8.3 15.1 18.9 27.2 30.1 35.5-20.8 4.3-38.9 13.1-54.8 19.2zm131.6-5s-5 6-37.3-7.8c35.1-2.6 40.9 5.4 37.3 7.8z"></path></svg> `\(~~~\)` **Peer review** <svg viewBox="0 0 512 512" style="position:relative;display:inline-block;top:.1em;fill:#035AA6;height:1em;" xmlns="http://www.w3.org/2000/svg"> <path d="M505 174.8l-39.6-39.6c-9.4-9.4-24.6-9.4-33.9 0L192 374.7 80.6 263.2c-9.4-9.4-24.6-9.4-33.9 0L7 302.9c-9.4 9.4-9.4 24.6 0 34L175 505c9.4 9.4 24.6 9.4 33.9 0l296-296.2c9.4-9.5 9.4-24.7.1-34zm-324.3 106c6.2 6.3 16.4 6.3 22.6 0l208-208.2c6.2-6.3 6.2-16.4 0-22.6L366.1 4.7c-6.2-6.3-16.4-6.3-22.6 0L192 156.2l-55.4-55.5c-6.2-6.3-16.4-6.3-22.6 0L68.7 146c-6.2 6.3-6.2 16.4 0 22.6l112 112.2z"></path></svg> `\(~~\)` **Replication** ] ] .pull-right[ ] --- background-image: url("talk/meteor.jpeg") background-position: 90% 73% background-size: 42% .center[ # Crowd Science ] ### The organization of scientific research in open and collaborative projects From highly **inclusive** (sourcing citizens) to highly **selective** (sourcing specialists) Used at different **stages** of the research process .pull-left[ .pull-left[ <svg viewBox="0 0 512 512" style="position:relative;display:inline-block;top:.1em;fill:#035AA6;height:1em;" xmlns="http://www.w3.org/2000/svg"> <path d="M0 405.3V448c0 35.3 86 64 192 64s192-28.7 192-64v-42.7C342.7 434.4 267.2 448 192 448S41.3 434.4 0 405.3zM320 128c106 0 192-28.7 192-64S426 0 320 0 128 28.7 128 64s86 64 192 64zM0 300.4V352c0 35.3 86 64 192 64s192-28.7 192-64v-51.6c-41.3 34-116.9 51.6-192 51.6S41.3 334.4 0 300.4zm416 11c57.3-11.1 96-31.7 96-55.4v-42.7c-23.2 16.4-57.3 27.6-96 34.5v63.6zM192 160C86 160 0 195.8 0 240s86 80 192 80 192-35.8 192-80-86-80-192-80zm219.3 56.3c60-10.8 100.7-32 100.7-56.3v-42.7c-35.5 25.1-96.5 38.6-160.7 41.8 29.5 14.3 51.2 33.5 60 57.2z"></path></svg> `\(~~~\)` **Funding** <svg viewBox="0 0 352 512" style="position:relative;display:inline-block;top:.1em;fill:#035AA6;height:1em;" xmlns="http://www.w3.org/2000/svg"> <path d="M176 80c-52.94 0-96 43.06-96 96 0 8.84 7.16 16 16 16s16-7.16 16-16c0-35.3 28.72-64 64-64 8.84 0 16-7.16 16-16s-7.16-16-16-16zM96.06 459.17c0 3.15.93 6.22 2.68 8.84l24.51 36.84c2.97 4.46 7.97 7.14 13.32 7.14h78.85c5.36 0 10.36-2.68 13.32-7.14l24.51-36.84c1.74-2.62 2.67-5.7 2.68-8.84l.05-43.18H96.02l.04 43.18zM176 0C73.72 0 0 82.97 0 176c0 44.37 16.45 84.85 43.56 115.78 16.64 18.99 42.74 58.8 52.42 92.16v.06h48v-.12c-.01-4.77-.72-9.51-2.15-14.07-5.59-17.81-22.82-64.77-62.17-109.67-20.54-23.43-31.52-53.15-31.61-84.14-.2-73.64 59.67-128 127.95-128 70.58 0 128 57.42 128 128 0 30.97-11.24 60.85-31.65 84.14-39.11 44.61-56.42 91.47-62.1 109.46a47.507 47.507 0 0 0-2.22 14.3v.1h48v-.05c9.68-33.37 35.78-73.18 52.42-92.16C335.55 260.85 352 220.37 352 176 352 78.8 273.2 0 176 0z"></path></svg> `\(~~~~\)` **Ideation** <svg viewBox="0 0 640 512" style="position:relative;display:inline-block;top:.1em;fill:#035AA6;height:1em;" xmlns="http://www.w3.org/2000/svg"> <path d="M384 320H256c-17.67 0-32 14.33-32 32v128c0 17.67 14.33 32 32 32h128c17.67 0 32-14.33 32-32V352c0-17.67-14.33-32-32-32zM192 32c0-17.67-14.33-32-32-32H32C14.33 0 0 14.33 0 32v128c0 17.67 14.33 32 32 32h95.72l73.16 128.04C211.98 300.98 232.4 288 256 288h.28L192 175.51V128h224V64H192V32zM608 0H480c-17.67 0-32 14.33-32 32v128c0 17.67 14.33 32 32 32h128c17.67 0 32-14.33 32-32V32c0-17.67-14.33-32-32-32z"></path></svg> `\(~~\)` **Study design** <svg viewBox="0 0 576 512" style="position:relative;display:inline-block;top:.1em;fill:#035AA6;height:1em;" xmlns="http://www.w3.org/2000/svg"> <path d="M527.9 224H480v-48c0-26.5-21.5-48-48-48H272l-64-64H48C21.5 64 0 85.5 0 112v288c0 26.5 21.5 48 48 48h400c16.5 0 31.9-8.5 40.7-22.6l79.9-128c20-31.9-3-73.4-40.7-73.4zM48 118c0-3.3 2.7-6 6-6h134.1l64 64H426c3.3 0 6 2.7 6 6v42H152c-16.8 0-32.4 8.8-41.1 23.2L48 351.4zm400 282H72l77.2-128H528z"></path></svg> `\(~~\)` **Data collection** ] .pull-right[ <svg viewBox="0 0 640 512" style="position:relative;display:inline-block;top:.1em;fill:#035AA6;height:1em;" xmlns="http://www.w3.org/2000/svg"> <path d="M255.03 261.65c6.25 6.25 16.38 6.25 22.63 0l11.31-11.31c6.25-6.25 6.25-16.38 0-22.63L253.25 192l35.71-35.72c6.25-6.25 6.25-16.38 0-22.63l-11.31-11.31c-6.25-6.25-16.38-6.25-22.63 0l-58.34 58.34c-6.25 6.25-6.25 16.38 0 22.63l58.35 58.34zm96.01-11.3l11.31 11.31c6.25 6.25 16.38 6.25 22.63 0l58.34-58.34c6.25-6.25 6.25-16.38 0-22.63l-58.34-58.34c-6.25-6.25-16.38-6.25-22.63 0l-11.31 11.31c-6.25 6.25-6.25 16.38 0 22.63L386.75 192l-35.71 35.72c-6.25 6.25-6.25 16.38 0 22.63zM624 416H381.54c-.74 19.81-14.71 32-32.74 32H288c-18.69 0-33.02-17.47-32.77-32H16c-8.8 0-16 7.2-16 16v16c0 35.2 28.8 64 64 64h512c35.2 0 64-28.8 64-64v-16c0-8.8-7.2-16-16-16zM576 48c0-26.4-21.6-48-48-48H112C85.6 0 64 21.6 64 48v336h512V48zm-64 272H128V64h384v256z"></path></svg> `\(~~\)` **Data analysis** <svg viewBox="0 0 512 512" style="position:relative;display:inline-block;top:.1em;fill:#035AA6;height:1em;" xmlns="http://www.w3.org/2000/svg"> <path d="M512 0C460.22 3.56 96.44 38.2 71.01 287.61c-3.09 26.66-4.84 53.44-5.99 80.24l178.87-178.69c6.25-6.25 16.4-6.25 22.65 0s6.25 16.38 0 22.63L7.04 471.03c-9.38 9.37-9.38 24.57 0 33.94 9.38 9.37 24.59 9.37 33.98 0l57.13-57.07c42.09-.14 84.15-2.53 125.96-7.36 53.48-5.44 97.02-26.47 132.58-56.54H255.74l146.79-48.88c11.25-14.89 21.37-30.71 30.45-47.12h-81.14l106.54-53.21C500.29 132.86 510.19 26.26 512 0z"></path></svg> `\(~~~\)` **Writing** <svg viewBox="0 0 384 512" style="position:relative;display:inline-block;top:.1em;fill:#035AA6;height:1em;" xmlns="http://www.w3.org/2000/svg"> <path d="M369.9 97.9L286 14C277 5 264.8-.1 252.1-.1H48C21.5 0 0 21.5 0 48v416c0 26.5 21.5 48 48 48h288c26.5 0 48-21.5 48-48V131.9c0-12.7-5.1-25-14.1-34zM332.1 128H256V51.9l76.1 76.1zM48 464V48h160v104c0 13.3 10.7 24 24 24h104v288H48zm250.2-143.7c-12.2-12-47-8.7-64.4-6.5-17.2-10.5-28.7-25-36.8-46.3 3.9-16.1 10.1-40.6 5.4-56-4.2-26.2-37.8-23.6-42.6-5.9-4.4 16.1-.4 38.5 7 67.1-10 23.9-24.9 56-35.4 74.4-20 10.3-47 26.2-51 46.2-3.3 15.8 26 55.2 76.1-31.2 22.4-7.4 46.8-16.5 68.4-20.1 18.9 10.2 41 17 55.8 17 25.5 0 28-28.2 17.5-38.7zm-198.1 77.8c5.1-13.7 24.5-29.5 30.4-35-19 30.3-30.4 35.7-30.4 35zm81.6-190.6c7.4 0 6.7 32.1 1.8 40.8-4.4-13.9-4.3-40.8-1.8-40.8zm-24.4 136.6c9.7-16.9 18-37 24.7-54.7 8.3 15.1 18.9 27.2 30.1 35.5-20.8 4.3-38.9 13.1-54.8 19.2zm131.6-5s-5 6-37.3-7.8c35.1-2.6 40.9 5.4 37.3 7.8z"></path></svg> `\(~~~\)` **Peer review** <svg viewBox="0 0 512 512" style="position:relative;display:inline-block;top:.1em;fill:#035AA6;height:1em;" xmlns="http://www.w3.org/2000/svg"> <path d="M505 174.8l-39.6-39.6c-9.4-9.4-24.6-9.4-33.9 0L192 374.7 80.6 263.2c-9.4-9.4-24.6-9.4-33.9 0L7 302.9c-9.4 9.4-9.4 24.6 0 34L175 505c9.4 9.4 24.6 9.4 33.9 0l296-296.2c9.4-9.5 9.4-24.7.1-34zm-324.3 106c6.2 6.3 16.4 6.3 22.6 0l208-208.2c6.2-6.3 6.2-16.4 0-22.6L366.1 4.7c-6.2-6.3-16.4-6.3-22.6 0L192 156.2l-55.4-55.5c-6.2-6.3-16.4-6.3-22.6 0L68.7 146c-6.2 6.3-6.2 16.4 0 22.6l112 112.2z"></path></svg> `\(~~\)` **Replication** ] ] .pull-right[ ] .right[ `\(~~\)` `\(~~\)` The great meteor storm of 1833: birth of *Citizen Science* ] ??? In 1833, Denison Olmsted used letter correspondence to recruit citizen scientists to help document a meteor shower. This led to a detailed documentation of the great meteor shower and the birth of the citizen-science movement (highly inclusive, used for data collection. In terms of the two dimensions we just discussed, this project can be categorized as highly inclusive - because it sourced citizens - and as used in the stage of data collection. --- class: inverse, center, middle name: crowddata # Crowdsourcing Data Analysis --- .center[ # Crowdsourced Data Analysis ] ??? Involves giving different teams of scientists the same dataset, who independently analyze it to answer the same research question or estimate and report on a parameter of interest. -- ### Different teams of scientists independently analyze the same dataset -- <svg viewBox="0 0 640 512" style="position:relative;display:inline-block;top:.1em;fill:#035AA6;height:2em;" xmlns="http://www.w3.org/2000/svg"> <path d="M96 224c35.3 0 64-28.7 64-64s-28.7-64-64-64-64 28.7-64 64 28.7 64 64 64zm448 0c35.3 0 64-28.7 64-64s-28.7-64-64-64-64 28.7-64 64 28.7 64 64 64zm32 32h-64c-17.6 0-33.5 7.1-45.1 18.6 40.3 22.1 68.9 62 75.1 109.4h66c17.7 0 32-14.3 32-32v-32c0-35.3-28.7-64-64-64zm-256 0c61.9 0 112-50.1 112-112S381.9 32 320 32 208 82.1 208 144s50.1 112 112 112zm76.8 32h-8.3c-20.8 10-43.9 16-68.5 16s-47.6-6-68.5-16h-8.3C179.6 288 128 339.6 128 403.2V432c0 26.5 21.5 48 48 48h288c26.5 0 48-21.5 48-48v-28.8c0-63.6-51.6-115.2-115.2-115.2zm-223.7-13.4C161.5 263.1 145.6 256 128 256H64c-35.3 0-64 28.7-64 64v32c0 17.7 14.3 32 32 32h65.9c6.3-47.4 34.9-87.3 75.2-109.4z"></path></svg> `\(~~\)` Also known as the **many-analysts** or **multi-analyst** approach -- <svg viewBox="0 0 512 512" style="position:relative;display:inline-block;top:.1em;fill:#035AA6;height:2em;" xmlns="http://www.w3.org/2000/svg"> <path d="M496 384H64V80c0-8.84-7.16-16-16-16H16C7.16 64 0 71.16 0 80v336c0 17.67 14.33 32 32 32h464c8.84 0 16-7.16 16-16v-32c0-8.84-7.16-16-16-16zM464 96H345.94c-21.38 0-32.09 25.85-16.97 40.97l32.4 32.4L288 242.75l-73.37-73.37c-12.5-12.5-32.76-12.5-45.25 0l-68.69 68.69c-6.25 6.25-6.25 16.38 0 22.63l22.62 22.62c6.25 6.25 16.38 6.25 22.63 0L192 237.25l73.37 73.37c12.5 12.5 32.76 12.5 45.25 0l96-96 32.4 32.4c15.12 15.12 40.97 4.41 40.97-16.97V112c.01-8.84-7.15-16-15.99-16z"></path></svg> `\(~~~~\)` On the **rise** in the social and behavioral sciences --
??? I'm including an interactive table with 7 papers that have recently been published in the social and behavioral science that use the many-analyst approach. They include interesting topics such as variability in replications, opinions about different social policies, the effects of the gender and professional status of scientists on their verbosity during group meetings, variability in the analysis of fmri (so neuroimaging datasets), and we can scroll back all the way to 2018, to a quite well-known study that looked at discrimination against darker skin toned soccer players, and arguably started the recent crowd science movement in behavioral science. --- # The Rationale of Crowdsourced Data Analysis ??? What is the rationale of crowdsourced data analysis - why should we be convinced by its findings? The phenomenon that underlies crowd science in general is known as the wisdom of the crowd. -- ### **The Wisdom of the Crowd** -- <svg viewBox="0 0 576 512" style="position:relative;display:inline-block;top:.1em;fill:#035AA6;height:1.5em;" xmlns="http://www.w3.org/2000/svg"> <path d="M215.03 71.05L126.06 160H24c-13.26 0-24 10.74-24 24v144c0 13.25 10.74 24 24 24h102.06l88.97 88.95c15.03 15.03 40.97 4.47 40.97-16.97V88.02c0-21.46-25.96-31.98-40.97-16.97zm233.32-51.08c-11.17-7.33-26.18-4.24-33.51 6.95-7.34 11.17-4.22 26.18 6.95 33.51 66.27 43.49 105.82 116.6 105.82 195.58 0 78.98-39.55 152.09-105.82 195.58-11.17 7.32-14.29 22.34-6.95 33.5 7.04 10.71 21.93 14.56 33.51 6.95C528.27 439.58 576 351.33 576 256S528.27 72.43 448.35 19.97zM480 256c0-63.53-32.06-121.94-85.77-156.24-11.19-7.14-26.03-3.82-33.12 7.46s-3.78 26.21 7.41 33.36C408.27 165.97 432 209.11 432 256s-23.73 90.03-63.48 115.42c-11.19 7.14-14.5 22.07-7.41 33.36 6.51 10.36 21.12 15.14 33.12 7.46C447.94 377.94 480 319.54 480 256zm-141.77-76.87c-11.58-6.33-26.19-2.16-32.61 9.45-6.39 11.61-2.16 26.2 9.45 32.61C327.98 228.28 336 241.63 336 256c0 14.38-8.02 27.72-20.92 34.81-11.61 6.41-15.84 21-9.45 32.61 6.43 11.66 21.05 15.8 32.61 9.45 28.23-15.55 45.77-45 45.77-76.88s-17.54-61.32-45.78-76.86z"></path></svg> `\(~~~\)` Individual judgments are associated with **idiosyncratic noise** -- <svg viewBox="0 0 640 512" style="position:relative;display:inline-block;top:.1em;fill:#035AA6;height:1.5em;" xmlns="http://www.w3.org/2000/svg"> <path d="M633.82 458.1L476.26 336.33C488.74 312.21 496 284.98 496 256v-48c0-8.84-7.16-16-16-16h-16c-8.84 0-16 7.16-16 16v48c0 17.92-3.96 34.8-10.72 50.2l-26.55-20.52c3.1-9.4 5.28-19.22 5.28-29.67h-43.67l-41.4-32H416v-32h-85.33c-5.89 0-10.67-3.58-10.67-8v-16c0-4.42 4.78-8 10.67-8H416v-32h-85.33c-5.89 0-10.67-3.58-10.67-8v-16c0-4.42 4.78-8 10.67-8H416c0-53.02-42.98-96-96-96s-96 42.98-96 96v45.36L45.47 3.37C38.49-2.05 28.43-.8 23.01 6.18L3.37 31.45C-2.05 38.42-.8 48.47 6.18 53.9l588.36 454.73c6.98 5.43 17.03 4.17 22.46-2.81l19.64-25.27c5.41-6.97 4.16-17.02-2.82-22.45zM400 464h-56v-33.78c11.71-1.62 23.1-4.28 33.96-8.08l-50.4-38.96c-6.71.4-13.41.87-20.35.2-55.85-5.45-98.74-48.63-111.18-101.85L144 241.31v6.85c0 89.64 63.97 169.55 152 181.69V464h-56c-8.84 0-16 7.16-16 16v16c0 8.84 7.16 16 16 16h160c8.84 0 16-7.16 16-16v-16c0-8.84-7.16-16-16-16z"></path></svg> `\(~~\)` Averaging over many judgments can **cancel** some of that noise --- background-image: url("talk/ox.png") background-position: 99% 60% background-size: 35% # The Rationale of Crowdsourced Data Analysis ### **The Wisdom of the Crowd** <svg viewBox="0 0 576 512" style="position:relative;display:inline-block;top:.1em;fill:#035AA6;height:1.5em;" xmlns="http://www.w3.org/2000/svg"> <path d="M215.03 71.05L126.06 160H24c-13.26 0-24 10.74-24 24v144c0 13.25 10.74 24 24 24h102.06l88.97 88.95c15.03 15.03 40.97 4.47 40.97-16.97V88.02c0-21.46-25.96-31.98-40.97-16.97zm233.32-51.08c-11.17-7.33-26.18-4.24-33.51 6.95-7.34 11.17-4.22 26.18 6.95 33.51 66.27 43.49 105.82 116.6 105.82 195.58 0 78.98-39.55 152.09-105.82 195.58-11.17 7.32-14.29 22.34-6.95 33.5 7.04 10.71 21.93 14.56 33.51 6.95C528.27 439.58 576 351.33 576 256S528.27 72.43 448.35 19.97zM480 256c0-63.53-32.06-121.94-85.77-156.24-11.19-7.14-26.03-3.82-33.12 7.46s-3.78 26.21 7.41 33.36C408.27 165.97 432 209.11 432 256s-23.73 90.03-63.48 115.42c-11.19 7.14-14.5 22.07-7.41 33.36 6.51 10.36 21.12 15.14 33.12 7.46C447.94 377.94 480 319.54 480 256zm-141.77-76.87c-11.58-6.33-26.19-2.16-32.61 9.45-6.39 11.61-2.16 26.2 9.45 32.61C327.98 228.28 336 241.63 336 256c0 14.38-8.02 27.72-20.92 34.81-11.61 6.41-15.84 21-9.45 32.61 6.43 11.66 21.05 15.8 32.61 9.45 28.23-15.55 45.77-45 45.77-76.88s-17.54-61.32-45.78-76.86z"></path></svg> `\(~~~\)` Individual judgments are associated with **idiosyncratic noise** <svg viewBox="0 0 640 512" style="position:relative;display:inline-block;top:.1em;fill:#035AA6;height:1.5em;" xmlns="http://www.w3.org/2000/svg"> <path d="M633.82 458.1L476.26 336.33C488.74 312.21 496 284.98 496 256v-48c0-8.84-7.16-16-16-16h-16c-8.84 0-16 7.16-16 16v48c0 17.92-3.96 34.8-10.72 50.2l-26.55-20.52c3.1-9.4 5.28-19.22 5.28-29.67h-43.67l-41.4-32H416v-32h-85.33c-5.89 0-10.67-3.58-10.67-8v-16c0-4.42 4.78-8 10.67-8H416v-32h-85.33c-5.89 0-10.67-3.58-10.67-8v-16c0-4.42 4.78-8 10.67-8H416c0-53.02-42.98-96-96-96s-96 42.98-96 96v45.36L45.47 3.37C38.49-2.05 28.43-.8 23.01 6.18L3.37 31.45C-2.05 38.42-.8 48.47 6.18 53.9l588.36 454.73c6.98 5.43 17.03 4.17 22.46-2.81l19.64-25.27c5.41-6.97 4.16-17.02-2.82-22.45zM400 464h-56v-33.78c11.71-1.62 23.1-4.28 33.96-8.08l-50.4-38.96c-6.71.4-13.41.87-20.35.2-55.85-5.45-98.74-48.63-111.18-101.85L144 241.31v6.85c0 89.64 63.97 169.55 152 181.69V464h-56c-8.84 0-16 7.16-16 16v16c0 8.84 7.16 16 16 16h160c8.84 0 16-7.16 16-16v-16c0-8.84-7.16-16-16-16z"></path></svg> `\(~~\)` Averaging over many judgments can **cancel** some of that noise `\(~~\)` In 1906, 787 people guessed the weight of an ox at a farmers' fair... <svg viewBox="0 0 256 512" style="position:relative;display:inline-block;top:.1em;fill:#035AA6;height:1.5em;" xmlns="http://www.w3.org/2000/svg"> <path d="M224.3 273l-136 136c-9.4 9.4-24.6 9.4-33.9 0l-22.6-22.6c-9.4-9.4-9.4-24.6 0-33.9l96.4-96.4-96.4-96.4c-9.4-9.4-9.4-24.6 0-33.9L54.3 103c9.4-9.4 24.6-9.4 33.9 0l136 136c9.5 9.4 9.5 24.6.1 34z"></path></svg> `\(~~\)` The **mean** of all guesses was exactly **equal to the true value** <svg viewBox="0 0 256 512" style="position:relative;display:inline-block;top:.1em;fill:#035AA6;height:1.5em;" xmlns="http://www.w3.org/2000/svg"> <path d="M224.3 273l-136 136c-9.4 9.4-24.6 9.4-33.9 0l-22.6-22.6c-9.4-9.4-9.4-24.6 0-33.9l96.4-96.4-96.4-96.4c-9.4-9.4-9.4-24.6 0-33.9L54.3 103c9.4-9.4 24.6-9.4 33.9 0l136 136c9.5 9.4 9.5 24.6.1 34z"></path></svg> `\(~~\)` The mean **outperformed** the estimates of **experts** <svg viewBox="0 0 256 512" style="position:relative;display:inline-block;top:.1em;fill:#035AA6;height:1.5em;" xmlns="http://www.w3.org/2000/svg"> <path d="M224.3 273l-136 136c-9.4 9.4-24.6 9.4-33.9 0l-22.6-22.6c-9.4-9.4-9.4-24.6 0-33.9l96.4-96.4-96.4-96.4c-9.4-9.4-9.4-24.6 0-33.9L54.3 103c9.4-9.4 24.6-9.4 33.9 0l136 136c9.5 9.4 9.5 24.6.1 34z"></path></svg> `\(~~\)` The mean **outperformed** the individual **best guesser** ??? ??? Perhaps the first documented case of the wisdom of the crowd was back in 1906, when... 1197 pounds, cattle experts, individual best guesser who took home the grand prize that day. This may seem unsurprising now, but it wasn't back then. Back then, the fact that crowds were wise was a surprise. Crowds mostly had negative associations, were referred to as mobs, and there was heated intellectual debate about restricting the right to vote to intellectuals, rather than democratizing society. To benefit from the wisdom of crowds several conditions must be in place. First each individual member of the crowd must have their own independent source of information. Second they must make individual decisions and not be swayed by the decisions of those around them. And third, there must be a mechanism in place that can collate these diverse opinions. --- # Benefits of Crowdsourced Data Analysis ### **Crowdsourced data analysis can help to...** ??? I'll mention some benefits, some of which will overlap, and most of them are intimately related with each other. -- <svg viewBox="0 0 640 512" style="position:relative;display:inline-block;top:.1em;fill:#035AA6;height:1.5em;" xmlns="http://www.w3.org/2000/svg"> <path d="M96 224c35.3 0 64-28.7 64-64s-28.7-64-64-64-64 28.7-64 64 28.7 64 64 64zm448 0c35.3 0 64-28.7 64-64s-28.7-64-64-64-64 28.7-64 64 28.7 64 64 64zm32 32h-64c-17.6 0-33.5 7.1-45.1 18.6 40.3 22.1 68.9 62 75.1 109.4h66c17.7 0 32-14.3 32-32v-32c0-35.3-28.7-64-64-64zm-256 0c61.9 0 112-50.1 112-112S381.9 32 320 32 208 82.1 208 144s50.1 112 112 112zm76.8 32h-8.3c-20.8 10-43.9 16-68.5 16s-47.6-6-68.5-16h-8.3C179.6 288 128 339.6 128 403.2V432c0 26.5 21.5 48 48 48h288c26.5 0 48-21.5 48-48v-28.8c0-63.6-51.6-115.2-115.2-115.2zm-223.7-13.4C161.5 263.1 145.6 256 128 256H64c-35.3 0-64 28.7-64 64v32c0 17.7 14.3 32 32 32h65.9c6.3-47.4 34.9-87.3 75.2-109.4z"></path></svg> `\(~~\)` **Diversify** contributions to research ??? First, crowdsourced data analysis can help to diversify contributions to research. I will say that this benefit is not specific to crowdsourcing data analysis, but a property of crowd science and big team science in general. A goal of crowd science and big team science is to promote inclusivity and include researchers from underrepresented institutions. This comes with all the benefits of diversity. It allows us to leverage under-used resources and skills. We of course can't all be an expert on everything. If we recruit a diverse sample of data analysts, some may have expertise, for example, on a certain statistical method that is most appropriate for the data at hand, or they'll have some other expertise that may benefit the data analysis. -- <svg viewBox="0 0 640 512" style="position:relative;display:inline-block;top:.1em;fill:#035AA6;height:1.5em;" xmlns="http://www.w3.org/2000/svg"> <path d="M368 32h-96c-17.67 0-32 14.33-32 32v96c0 17.67 14.33 32 32 32h96c17.67 0 32-14.33 32-32V64c0-17.67-14.33-32-32-32zM208 88h-84.75C113.75 64.56 90.84 48 64 48 28.66 48 0 76.65 0 112s28.66 64 64 64c26.84 0 49.75-16.56 59.25-40h79.73c-55.37 32.52-95.86 87.32-109.54 152h49.4c11.3-41.61 36.77-77.21 71.04-101.56-3.7-8.08-5.88-16.99-5.88-26.44V88zm-48 232H64c-17.67 0-32 14.33-32 32v96c0 17.67 14.33 32 32 32h96c17.67 0 32-14.33 32-32v-96c0-17.67-14.33-32-32-32zM576 48c-26.84 0-49.75 16.56-59.25 40H432v72c0 9.45-2.19 18.36-5.88 26.44 34.27 24.35 59.74 59.95 71.04 101.56h49.4c-13.68-64.68-54.17-119.48-109.54-152h79.73c9.5 23.44 32.41 40 59.25 40 35.34 0 64-28.65 64-64s-28.66-64-64-64zm0 272h-96c-17.67 0-32 14.33-32 32v96c0 17.67 14.33 32 32 32h96c17.67 0 32-14.33 32-32v-96c0-17.67-14.33-32-32-32z"></path></svg> `\(~~\)` Expose the **inherent variability** of results ??? Second, the many-analyst approach helps to acknowledge that results in social science tend to be very variable, and can depend on idiosyncratic choices we have to make among multiple valid approaches, sometimes even choices we make unconsciously, such as the version of statistical software we rely on or the different defaults in for instance SPSS and R: it is important to note that the many-analyst approach does not *create* this variability in results, it simply *exposes* it. that should give us pause and epistemic humility when we assess conventional scientific research, which is often done by a single analyst or a single team: the particular outcome you're looking at is one from a distribution of outcomes that could have been possible, if the analyst had made different choices. Like Nicholas said in his opening talk, approaches like this allow us to explore the size of the multiverse. -- <svg viewBox="0 0 512 512" style="position:relative;display:inline-block;top:.1em;fill:#035AA6;height:1.5em;" xmlns="http://www.w3.org/2000/svg"> <path d="M416 320h-96c-17.6 0-32-14.4-32-32s14.4-32 32-32h96s96-107 96-160-43-96-96-96-96 43-96 96c0 25.5 22.2 63.4 45.3 96H320c-52.9 0-96 43.1-96 96s43.1 96 96 96h96c17.6 0 32 14.4 32 32s-14.4 32-32 32H185.5c-16 24.8-33.8 47.7-47.3 64H416c52.9 0 96-43.1 96-96s-43.1-96-96-96zm0-256c17.7 0 32 14.3 32 32s-14.3 32-32 32-32-14.3-32-32 14.3-32 32-32zM96 256c-53 0-96 43-96 96s96 160 96 160 96-107 96-160-43-96-96-96zm0 128c-17.7 0-32-14.3-32-32s14.3-32 32-32 32 14.3 32 32-14.3 32-32 32z"></path></svg> `\(~~~\)` Assess the **impact of individual analytic choices** on the results ??? Third, we can assess the impact of individual analytic choices on the results. Here, we can ask if we can explain the strength of the evidence obtained by the specific individual decisions that researchers make. For instance, we can asses the impact of including or excluding certain control variables, the impact of running a certain statistical model such as a t-test or a mixed effects model, or the impact of excluding or including certain participants or outliers. We could ask, for example, if people who obtain stronger evidence are more likely to do one or the other, and vice versa. -- <svg viewBox="0 0 640 512" style="position:relative;display:inline-block;top:.1em;fill:#035AA6;height:1.4em;" xmlns="http://www.w3.org/2000/svg"> <path d="M104 96H56c-13.3 0-24 10.7-24 24v104H8c-4.4 0-8 3.6-8 8v48c0 4.4 3.6 8 8 8h24v104c0 13.3 10.7 24 24 24h48c13.3 0 24-10.7 24-24V120c0-13.3-10.7-24-24-24zm528 128h-24V120c0-13.3-10.7-24-24-24h-48c-13.3 0-24 10.7-24 24v272c0 13.3 10.7 24 24 24h48c13.3 0 24-10.7 24-24V288h24c4.4 0 8-3.6 8-8v-48c0-4.4-3.6-8-8-8zM456 32h-48c-13.3 0-24 10.7-24 24v168H256V56c0-13.3-10.7-24-24-24h-48c-13.3 0-24 10.7-24 24v400c0 13.3 10.7 24 24 24h48c13.3 0 24-10.7 24-24V288h128v168c0 13.3 10.7 24 24 24h48c13.3 0 24-10.7 24-24V56c0-13.3-10.7-24-24-24z"></path></svg> `\(~~\)` Assess the overall **analytic robustness** of a finding ??? Fourth, the many-analyst approach can help us assess the overall analytic robustness of a finding. We can assess to what extent a finding holds across many different analytic specification. We can ask: do the results come out consistently positive, or consistently negative, across different independent analyses? -- <svg viewBox="0 0 640 512" style="position:relative;display:inline-block;top:.1em;fill:#035AA6;height:1.5em;" xmlns="http://www.w3.org/2000/svg"> <path d="M72 64h24v240c0 44.1 35.9 80 80 80s80-35.9 80-80V64h24c4.4 0 8-3.6 8-8V8c0-4.4-3.6-8-8-8H72c-4.4 0-8 3.6-8 8v48c0 4.4 3.6 8 8 8zm72 0h64v96h-64V64zm480 384H16c-8.8 0-16 7.2-16 16v32c0 8.8 7.2 16 16 16h608c8.8 0 16-7.2 16-16v-32c0-8.8-7.2-16-16-16zM360 64h24v240c0 44.1 35.9 80 80 80s80-35.9 80-80V64h24c4.4 0 8-3.6 8-8V8c0-4.4-3.6-8-8-8H360c-4.4 0-8 3.6-8 8v48c0 4.4 3.6 8 8 8zm72 0h64v96h-64V64z"></path></svg> `\(~~\)` **Increase confidence** when independent analyses yield the same conclusion ??? And when we do find that the result come out consistently positive or negative, then this can increase our confidence in the specific conclusion we're drawing. -- <svg viewBox="0 0 448 512" style="position:relative;display:inline-block;top:.1em;fill:#035AA6;height:1.5em;" xmlns="http://www.w3.org/2000/svg"> <path d="M224 352c-35.35 0-64 28.65-64 64s28.65 64 64 64 64-28.65 64-64-28.65-64-64-64zm0-192c35.35 0 64-28.65 64-64s-28.65-64-64-64-64 28.65-64 64 28.65 64 64 64zm192 48H32c-17.67 0-32 14.33-32 32v32c0 17.67 14.33 32 32 32h384c17.67 0 32-14.33 32-32v-32c0-17.67-14.33-32-32-32z"></path></svg> `\(~~~~\)` Average across idiosyncratic analytic choices to obtain **more accurate parameter estimates** ??? And going back to the wisdom of the crowd phenomenon that we discussed, another benefit is that we can average across all these idiosyncratic analytic choices to obtain more accurate parameter estimates and to balance out individual biases and errors in the aggregate -- <svg viewBox="0 0 640 512" style="position:relative;display:inline-block;top:.1em;fill:#035AA6;height:1.5em;" xmlns="http://www.w3.org/2000/svg"> <path d="M256 336h-.02c0-16.18 1.34-8.73-85.05-181.51-17.65-35.29-68.19-35.36-85.87 0C-2.06 328.75.02 320.33.02 336H0c0 44.18 57.31 80 128 80s128-35.82 128-80zM128 176l72 144H56l72-144zm511.98 160c0-16.18 1.34-8.73-85.05-181.51-17.65-35.29-68.19-35.36-85.87 0-87.12 174.26-85.04 165.84-85.04 181.51H384c0 44.18 57.31 80 128 80s128-35.82 128-80h-.02zM440 320l72-144 72 144H440zm88 128H352V153.25c23.51-10.29 41.16-31.48 46.39-57.25H528c8.84 0 16-7.16 16-16V48c0-8.84-7.16-16-16-16H383.64C369.04 12.68 346.09 0 320 0s-49.04 12.68-63.64 32H112c-8.84 0-16 7.16-16 16v32c0 8.84 7.16 16 16 16h129.61c5.23 25.76 22.87 46.96 46.39 57.25V448H112c-8.84 0-16 7.16-16 16v32c0 8.84 7.16 16 16 16h416c8.84 0 16-7.16 16-16v-32c0-8.84-7.16-16-16-16z"></path></svg> `\(~~\)` ... and to **balance out individual biases and errors** in the aggregate --- class: inverse, center, middle name: lay # Lay Perceptions ??? Now I'd like to move on to talking about lay perceptions. One of the reasons crowdsourced data analysis was proposed, was to increase the credibility of scientific research. Now, we know there's quite a great deal of science skepticism in some public circles, so we want scientific research to become more credible not just to ourselves as academics, but to lay people as well. --- # Lay Perceptions of Crowd-Scientific Findings According to its proponents, crowdsourcing data analysis should **improve lay perceptions of scientific findings** --
??? I'm including here another table with three quotes. The first one is from a preregistration, in which the authors justify their use of the many-analyst approach by referring to the public needing to have faith in the conclusions of scientists. The second one is from a commentary on the many-analyst approach, in which the author provides the hopeful view that crowdsourcing provides a new way to increase credibility for social research, not just among researchers themselves, but in sample populations as well; and that it's hoped that the many-analyst approach will increase public views of social science. The third one is from a preprint relying on the many-analyst approach, in which the authors argue that many-analyst studies that generate consistent results across independent analysis should offer consumers of scientific findings assurance that they are not arbitrary flukes. --- class: inverse, center, middle # Does Crowdsourcing Data Analysis Improve the Credibility of Scientific Findings <svg viewBox="0 0 512 512" style="position:relative;display:inline-block;top:.1em;fill:white;height:1em;" xmlns="http://www.w3.org/2000/svg"> <path d="M256 8C119.043 8 8 119.083 8 256c0 136.997 111.043 248 248 248s248-111.003 248-248C504 119.083 392.957 8 256 8zm0 448c-110.532 0-200-89.431-200-200 0-110.495 89.472-200 200-200 110.491 0 200 89.471 200 200 0 110.53-89.431 200-200 200zm107.244-255.2c0 67.052-72.421 68.084-72.421 92.863V300c0 6.627-5.373 12-12 12h-45.647c-6.627 0-12-5.373-12-12v-8.659c0-35.745 27.1-50.034 47.579-61.516 17.561-9.845 28.324-16.541 28.324-29.579 0-17.246-21.999-28.693-39.784-28.693-23.189 0-33.894 10.977-48.942 29.969-4.057 5.12-11.46 6.071-16.666 2.124l-27.824-21.098c-5.107-3.872-6.251-11.066-2.644-16.363C184.846 131.491 214.94 112 261.794 112c49.071 0 101.45 38.304 101.45 88.8zM298 368c0 23.159-18.841 42-42 42s-42-18.841-42-42 18.841-42 42-42 42 18.841 42 42z"></path></svg> ??? Now, these quotes describe a worthy goal: improving the credibility of scientific findings through crowdsourcing data analysis. However, this is also an empirical claim, and as far as we know there is no evidence yet that crowdsourcing data analysis can indeed achieve this goal. So that's what we'll be looking at today: does crowdsourcing data analysis improve lay perceptions of scientific findings? --- class: inverse, center, middle name: method # Method --- # Method -- We run an experiment with **three conditions** ??? Three scenarios. The first condition is a conventional, single-analyst condition where a single team of six scientists analyzes the same dataset and reports a single, aggregrate parameter estimate of 5% for the relationship between two variables that I'll introduce shortly. -- <svg viewBox="0 0 448 512" style="position:relative;display:inline-block;top:.1em;fill:#035AA6;height:1.5em;" xmlns="http://www.w3.org/2000/svg"> <path d="M384 32H64C28.65 32 0 60.65 0 96v320c0 35.35 28.65 64 64 64h320c35.35 0 64-28.65 64-64V96c0-35.35-28.65-64-64-64zM224 288c-17.67 0-32-14.33-32-32s14.33-32 32-32 32 14.33 32 32-14.33 32-32 32z"></path></svg> `\(~~\)` **Single-analyst** `\(~~~~~~~~~~~\)` a *single, aggregate parameter estimate* ??? We contrast this condition with two multi-analyst conditions. In the multi-consistent conditon, six independent teams of scientists report six independent estimates that show low variance and high consensus: they all agree in their conclusion, and the spread in their effect size estimate is small. -- <svg viewBox="0 0 448 512" style="position:relative;display:inline-block;top:.1em;fill:#035AA6;height:1.5em;" xmlns="http://www.w3.org/2000/svg"> <path d="M384 32H64C28.65 32 0 60.65 0 96v320c0 35.35 28.65 64 64 64h320c35.35 0 64-28.65 64-64V96c0-35.35-28.65-64-64-64zM128 192c-17.67 0-32-14.33-32-32s14.33-32 32-32 32 14.33 32 32-14.33 32-32 32zm192 192c-17.67 0-32-14.33-32-32s14.33-32 32-32 32 14.33 32 32-14.33 32-32 32z"></path></svg> `\(~~\)` **Multi-consistent** `\(~~~~~~~\)` *multiple parameter estimates* with *low variance* and *high consensus* ??? In the multi-consistent condition, six independent teams of scientists report six independent estimates that show high variance and low consensus. There is a large spread between the estimates, and the qualitative conclusions differ: two of the scientists find a negative relationship, and four of the scientists find a positive relationship. -- <svg viewBox="0 0 448 512" style="position:relative;display:inline-block;top:.1em;fill:#035AA6;height:1.5em;" xmlns="http://www.w3.org/2000/svg"> <path d="M384 32H64C28.65 32 0 60.65 0 96v320c0 35.35 28.65 64 64 64h320c35.35 0 64-28.65 64-64V96c0-35.35-28.65-64-64-64zM128 192c-17.67 0-32-14.33-32-32s14.33-32 32-32 32 14.33 32 32-14.33 32-32 32zm96 96c-17.67 0-32-14.33-32-32s14.33-32 32-32 32 14.33 32 32-14.33 32-32 32zm96 96c-17.67 0-32-14.33-32-32s14.33-32 32-32 32 14.33 32 32-14.33 32-32 32z"></path></svg> `\(~~\)` **Multi-inconsistent** `\(~~~~\)` *multiple parameter estimates* with *high variance* and *low consensus* -- `\(~~\)` In all three conditions, the given estimates average to 5%. We study the **effects on** .pull-left[ <svg viewBox="0 0 256 512" style="position:relative;display:inline-block;top:.1em;fill:#035AA6;height:1.5em;" xmlns="http://www.w3.org/2000/svg"> <path d="M224.3 273l-136 136c-9.4 9.4-24.6 9.4-33.9 0l-22.6-22.6c-9.4-9.4-9.4-24.6 0-33.9l96.4-96.4-96.4-96.4c-9.4-9.4-9.4-24.6 0-33.9L54.3 103c9.4-9.4 24.6-9.4 33.9 0l136 136c9.5 9.4 9.5 24.6.1 34z"></path></svg> **Posterior beliefs** about the reported phenomenon <svg viewBox="0 0 256 512" style="position:relative;display:inline-block;top:.1em;fill:#035AA6;height:1.5em;" xmlns="http://www.w3.org/2000/svg"> <path d="M224.3 273l-136 136c-9.4 9.4-24.6 9.4-33.9 0l-22.6-22.6c-9.4-9.4-9.4-24.6 0-33.9l96.4-96.4-96.4-96.4c-9.4-9.4-9.4-24.6 0-33.9L54.3 103c9.4-9.4 24.6-9.4 33.9 0l136 136c9.5 9.4 9.5 24.6.1 34z"></path></svg> **Credibility** of the results <svg viewBox="0 0 256 512" style="position:relative;display:inline-block;top:.1em;fill:#035AA6;height:1.5em;" xmlns="http://www.w3.org/2000/svg"> <path d="M224.3 273l-136 136c-9.4 9.4-24.6 9.4-33.9 0l-22.6-22.6c-9.4-9.4-9.4-24.6 0-33.9l96.4-96.4-96.4-96.4c-9.4-9.4-9.4-24.6 0-33.9L54.3 103c9.4-9.4 24.6-9.4 33.9 0l136 136c9.5 9.4 9.5 24.6.1 34z"></path></svg> **Confidence** in the aggregate estimate of 5% ] .pull-right[ <svg viewBox="0 0 256 512" style="position:relative;display:inline-block;top:.1em;fill:#035AA6;height:1.5em;" xmlns="http://www.w3.org/2000/svg"> <path d="M224.3 273l-136 136c-9.4 9.4-24.6 9.4-33.9 0l-22.6-22.6c-9.4-9.4-9.4-24.6 0-33.9l96.4-96.4-96.4-96.4c-9.4-9.4-9.4-24.6 0-33.9L54.3 103c9.4-9.4 24.6-9.4 33.9 0l136 136c9.5 9.4 9.5 24.6.1 34z"></path></svg> Perceived impact of **bias** on the 5% estimate <svg viewBox="0 0 256 512" style="position:relative;display:inline-block;top:.1em;fill:#035AA6;height:1.5em;" xmlns="http://www.w3.org/2000/svg"> <path d="M224.3 273l-136 136c-9.4 9.4-24.6 9.4-33.9 0l-22.6-22.6c-9.4-9.4-9.4-24.6 0-33.9l96.4-96.4-96.4-96.4c-9.4-9.4-9.4-24.6 0-33.9L54.3 103c9.4-9.4 24.6-9.4 33.9 0l136 136c9.5 9.4 9.5 24.6.1 34z"></path></svg> Perceived impact of **error** on the 5% estimate <svg viewBox="0 0 256 512" style="position:relative;display:inline-block;top:.1em;fill:#035AA6;height:1.5em;" xmlns="http://www.w3.org/2000/svg"> <path d="M224.3 273l-136 136c-9.4 9.4-24.6 9.4-33.9 0l-22.6-22.6c-9.4-9.4-9.4-24.6 0-33.9l96.4-96.4-96.4-96.4c-9.4-9.4-9.4-24.6 0-33.9L54.3 103c9.4-9.4 24.6-9.4 33.9 0l136 136c9.5 9.4 9.5 24.6.1 34z"></path></svg> Perceived impact of **discretion** on the 5% estimate ] ??? In all three conditions the estimates average to 5% --- class: left # Experimental design -- <img src="talk/mermaid.png" width="95%" style="display: block; margin: auto;" /> ??? In terms of the experimental design, participants are first introduced to a research question - do religious people report higher well-being - and then report their prior beliefs about this research question. They are then allocated to one of the three conditions, and afterwards report the outcomes that I just described. --- .center[ # Hypotheses ] ### **Social norms theory** Observing consensus among a crowd increases **conformity** in opinion ??? We based our hypotheses on three lines of thought. First, in line with social norms theory, we expect that observing consensus among a crowd (the consistent crowd condition) will -- compared to the conclusion of a single scientist (the single estimate condition) -- increase conformity in opinion. -- ### **Intuitive statistics ** Observers intuitively accord to the logic of **the wisdom of crowds**: The ability of an aggregate of estimates to reduce noise stemming from individual bias or error ??? We based our hypotheses on three lines of thought. First, in line with social norms theory, we expect that observing consensus among a crowd (the consistent crowd condition) will -- compared to the conclusion of a single scientist (the single estimate condition) -- increase conformity in opinion. Second, Drawing from work on intuitive statistics, we also expect laypeople to intuitively accord to the logic of "the wisdom of crowds": the ability of an aggregate of estimates (rather than a single estimate) to reduce noise stemming from individual bias or error. In contrast, when crowd estimates show low consensus and high variance (the inconsistent crowd condition), we predict that observers will be less swayed and more likely to attribute the findings to bias and error. -- ### **Aversion to variability** Observing **variable estimates** decreases confidence in the overall estimate ??? We based our hypotheses on three lines of thought. First, in line with social norms theory, we expect that observing consensus among a crowd (the consistent crowd condition) will -- compared to the conclusion of a single scientist (the single estimate condition) -- increase conformity in opinion. Second, Drawing from work on intuitive statistics, we also expect laypeople to intuitively accord to the logic of "the wisdom of crowds": the ability of an aggregate of estimates (rather than a single estimate) to reduce noise stemming from individual bias or error. In contrast, when crowd estimates show low consensus and high variance (the inconsistent crowd condition), we predict that observers will be less swayed and more likely to attribute the findings to bias and error. Third, due to the difficulty of lay reasoning about variation, we predict an aversion to variability: i.e., we expect that observing variable estimates will decrease lay confidence in the precise average parameter estimate in both crowd conditions. This is based on the idea that it may be completely foreign to lay consumers that analyzing the dataset can lead to different estimates in the first place. If we report one estimate, this is taken to be the "one true" estimate -- if we report several variable estimates, the consumer in question may no longer be so certain that any of the estimates, or the overall average estimate, is precisely correct. In sum, our preregistered hypotheses are as follows: when laypeople observe multiple consistent estimates from a crowd of independent scientists, we expect -- compared to a single estimate and controlling for prior beliefs -- higher posterior beliefs and credibility of the results, lower confidence in the precise average parameter estimate, and lower ratings of bias and error. When laypeople observe multiple inconsistent estimates from a crowd of independent scientists, we expect -- compared to a single estimate and controlling for prior beliefs -- lower posterior beliefs and credibility of the results, lower confidence in the precise average parameter estimate, and greater ratings of bias and error. --- .center[ # Hypotheses ] `\(~~\)` .pull-left[ <svg viewBox="0 0 496 512" style="position:relative;display:inline-block;top:.1em;fill:#035AA6;height:1.5em;" xmlns="http://www.w3.org/2000/svg"> <path d="M347.94 129.86L203.6 195.83a31.938 31.938 0 0 0-15.77 15.77l-65.97 144.34c-7.61 16.65 9.54 33.81 26.2 26.2l144.34-65.97a31.938 31.938 0 0 0 15.77-15.77l65.97-144.34c7.61-16.66-9.54-33.81-26.2-26.2zm-77.36 148.72c-12.47 12.47-32.69 12.47-45.16 0-12.47-12.47-12.47-32.69 0-45.16 12.47-12.47 32.69-12.47 45.16 0 12.47 12.47 12.47 32.69 0 45.16zM248 8C111.03 8 0 119.03 0 256s111.03 248 248 248 248-111.03 248-248S384.97 8 248 8zm0 448c-110.28 0-200-89.72-200-200S137.72 56 248 56s200 89.72 200 200-89.72 200-200 200z"></path></svg> `\(~~~~\)`**Predicted direction of effects** <svg viewBox="0 0 640 512" style="position:relative;display:inline-block;top:.1em;fill:#035AA6;height:1.5em;" xmlns="http://www.w3.org/2000/svg"> <path d="M132.65,212.32,36.21,137.78A63.4,63.4,0,0,0,32,160a63.84,63.84,0,0,0,100.65,52.32Zm40.44,62.28A63.79,63.79,0,0,0,128,256H64A64.06,64.06,0,0,0,0,320v32a32,32,0,0,0,32,32H97.91A146.62,146.62,0,0,1,173.09,274.6ZM544,224a64,64,0,1,0-64-64A64.06,64.06,0,0,0,544,224ZM500.56,355.11a114.24,114.24,0,0,0-84.47-65.28L361,247.23c41.46-16.3,71-55.92,71-103.23A111.93,111.93,0,0,0,320,32c-57.14,0-103.69,42.83-110.6,98.08L45.46,3.38A16,16,0,0,0,23,6.19L3.37,31.46A16,16,0,0,0,6.18,53.91L594.53,508.63A16,16,0,0,0,617,505.82l19.64-25.27a16,16,0,0,0-2.81-22.45ZM128,403.21V432a48,48,0,0,0,48,48H464a47.45,47.45,0,0,0,12.57-1.87L232,289.13C173.74,294.83,128,343.42,128,403.21ZM576,256H512a63.79,63.79,0,0,0-45.09,18.6A146.29,146.29,0,0,1,542,384h66a32,32,0,0,0,32-32V320A64.06,64.06,0,0,0,576,256Z"></path></svg> `\(~~~\)`**Compared to the single-analyst condition** <svg viewBox="0 0 512 512" style="position:relative;display:inline-block;top:.1em;fill:#035AA6;height:1.5em;" xmlns="http://www.w3.org/2000/svg"> <path d="M504 255.531c.253 136.64-111.18 248.372-247.82 248.468-59.015.042-113.223-20.53-155.822-54.911-11.077-8.94-11.905-25.541-1.839-35.607l11.267-11.267c8.609-8.609 22.353-9.551 31.891-1.984C173.062 425.135 212.781 440 256 440c101.705 0 184-82.311 184-184 0-101.705-82.311-184-184-184-48.814 0-93.149 18.969-126.068 49.932l50.754 50.754c10.08 10.08 2.941 27.314-11.313 27.314H24c-8.837 0-16-7.163-16-16V38.627c0-14.254 17.234-21.393 27.314-11.314l49.372 49.372C129.209 34.136 189.552 8 256 8c136.81 0 247.747 110.78 248 247.531zm-180.912 78.784l9.823-12.63c8.138-10.463 6.253-25.542-4.21-33.679L288 256.349V152c0-13.255-10.745-24-24-24h-16c-13.255 0-24 10.745-24 24v135.651l65.409 50.874c10.463 8.137 25.541 6.253 33.679-4.21z"></path></svg> `\(~~~~\)`**Controlling for prior beliefs** <svg viewBox="0 0 512 512" style="position:relative;display:inline-block;top:.1em;fill:#035AA6;height:1.5em;" xmlns="http://www.w3.org/2000/svg"> <g groupmode="layer" id="layer6" label="icon"> <path id="path246" style="fill-opacity:1;fill-rule:nonzero;stroke:none;stroke-width:0.0352777" d="m 255.96701,7.9999992 c -14.42056,0 -28.9134,3.1667198 -39.84197,9.5105508 L 69.402174,102.20596 c -21.954611,12.67476 -39.899208,43.75438 -39.899208,69.08441 v 169.41924 c 0,25.33653 17.944597,56.42568 39.899208,69.0939 L 216.12504,494.51788 C 227.05361,500.84232 241.54645,504 255.96701,504 c 14.48556,0 28.97013,-3.15768 39.89917,-9.48212 l 146.7224,-84.71437 c 21.92213,-12.66822 39.90845,-43.75737 39.90845,-69.0939 V 171.29037 c 0,-25.33003 -17.98632,-56.40965 -39.90845,-69.08441 L 295.86618,17.51055 C 284.93714,11.166719 270.45257,7.9999992 255.96701,7.9999992 Z M 355.1558,152.37339 c 4.22726,0 8.45019,1.60138 11.65118,4.81215 l 17.84669,17.82761 c 6.402,6.42793 6.402,16.93826 0,23.3597 L 228.22149,354.82393 c -6.46654,6.41499 -16.93871,6.41499 -23.34074,0 l -68.94211,-68.91386 c -6.40202,-6.41497 -5.044,-18.2773 0,-23.34073 l 17.85586,-17.82761 c 6.40202,-6.42793 16.9387,-6.42793 23.34073,0 l 27.74552,27.72655 c 6.40203,6.415 16.8742,6.415 23.34074,0 L 343.45702,157.18554 c 3.23307,-3.21072 7.47102,-4.81215 11.69878,-4.81215 z"></path> </g></svg> `\(~~~\)` [**Preregistration at osf.io/rpu98**](https://osf.io/rpu98) ] .pull-right[ | Measure | Multi-consistent | Multi-inconsistent | | ------------- | ------------- | ------------- | | Posterior beliefs | .center[<svg viewBox="0 0 640 512" style="position:relative;display:inline-block;top:.1em;fill:green;height:1.5em;" xmlns="http://www.w3.org/2000/svg"> <path d="M624 208h-64v-64c0-8.8-7.2-16-16-16h-32c-8.8 0-16 7.2-16 16v64h-64c-8.8 0-16 7.2-16 16v32c0 8.8 7.2 16 16 16h64v64c0 8.8 7.2 16 16 16h32c8.8 0 16-7.2 16-16v-64h64c8.8 0 16-7.2 16-16v-32c0-8.8-7.2-16-16-16zm-400 48c70.7 0 128-57.3 128-128S294.7 0 224 0 96 57.3 96 128s57.3 128 128 128zm89.6 32h-16.7c-22.2 10.2-46.9 16-72.9 16s-50.6-5.8-72.9-16h-16.7C60.2 288 0 348.2 0 422.4V464c0 26.5 21.5 48 48 48h352c26.5 0 48-21.5 48-48v-41.6c0-74.2-60.2-134.4-134.4-134.4z"></path></svg>] | .center[<svg viewBox="0 0 640 512" style="position:relative;display:inline-block;top:.1em;fill:red;height:1.5em;" xmlns="http://www.w3.org/2000/svg"> <path d="M624 208H432c-8.8 0-16 7.2-16 16v32c0 8.8 7.2 16 16 16h192c8.8 0 16-7.2 16-16v-32c0-8.8-7.2-16-16-16zm-400 48c70.7 0 128-57.3 128-128S294.7 0 224 0 96 57.3 96 128s57.3 128 128 128zm89.6 32h-16.7c-22.2 10.2-46.9 16-72.9 16s-50.6-5.8-72.9-16h-16.7C60.2 288 0 348.2 0 422.4V464c0 26.5 21.5 48 48 48h352c26.5 0 48-21.5 48-48v-41.6c0-74.2-60.2-134.4-134.4-134.4z"></path></svg>] | ] ??? *Note*. Table indicates the predicted direction of the effect for all dependent variables, compared to the single-analyst condition and controlling for prior beliefs. For example, we hypothesized that, compared to a single-analyst study and controlling for prior beliefs, ratings of credibility would be greater in the multi-analyst: consistent condition and lower in the multi-analyst: inconsistent condition. ??? We based our hypotheses on three lines of thought. First, in line with social norms theory, we expect that observing consensus among a crowd (the consistent crowd condition) will -- compared to the conclusion of a single scientist (the single estimate condition) -- increase conformity in opinion. Second, Drawing from work on intuitive statistics, we also expect laypeople to intuitively accord to the logic of "the wisdom of crowds": the ability of an aggregate of estimates (rather than a single estimate) to reduce noise stemming from individual bias or error. In contrast, when crowd estimates show low consensus and high variance (the inconsistent crowd condition), we predict that observers will be less swayed and more likely to attribute the findings to bias and error. Third, due to the difficulty of lay reasoning about variation, we predict an aversion to variability: i.e., we expect that observing variable estimates will decrease lay confidence in the precise average parameter estimate in both crowd conditions. In sum, our preregistered hypotheses are as follows: when laypeople observe multiple consistent estimates from a crowd of independent scientists, we expect -- compared to a single estimate and controlling for prior beliefs -- higher posterior beliefs and credibility of the results, lower confidence in the precise average parameter estimate, and lower ratings of bias and error. When laypeople observe multiple inconsistent estimates from a crowd of independent scientists, we expect -- compared to a single estimate and controlling for prior beliefs -- lower posterior beliefs and credibility of the results, lower confidence in the precise average parameter estimate, and greater ratings of bias and error. --- .center[ # Hypotheses ] `\(~~\)` .pull-left[ <svg viewBox="0 0 496 512" style="position:relative;display:inline-block;top:.1em;fill:#035AA6;height:1.5em;" xmlns="http://www.w3.org/2000/svg"> <path d="M347.94 129.86L203.6 195.83a31.938 31.938 0 0 0-15.77 15.77l-65.97 144.34c-7.61 16.65 9.54 33.81 26.2 26.2l144.34-65.97a31.938 31.938 0 0 0 15.77-15.77l65.97-144.34c7.61-16.66-9.54-33.81-26.2-26.2zm-77.36 148.72c-12.47 12.47-32.69 12.47-45.16 0-12.47-12.47-12.47-32.69 0-45.16 12.47-12.47 32.69-12.47 45.16 0 12.47 12.47 12.47 32.69 0 45.16zM248 8C111.03 8 0 119.03 0 256s111.03 248 248 248 248-111.03 248-248S384.97 8 248 8zm0 448c-110.28 0-200-89.72-200-200S137.72 56 248 56s200 89.72 200 200-89.72 200-200 200z"></path></svg> `\(~~~~\)`**Predicted direction of effects** <svg viewBox="0 0 640 512" style="position:relative;display:inline-block;top:.1em;fill:#035AA6;height:1.5em;" xmlns="http://www.w3.org/2000/svg"> <path d="M132.65,212.32,36.21,137.78A63.4,63.4,0,0,0,32,160a63.84,63.84,0,0,0,100.65,52.32Zm40.44,62.28A63.79,63.79,0,0,0,128,256H64A64.06,64.06,0,0,0,0,320v32a32,32,0,0,0,32,32H97.91A146.62,146.62,0,0,1,173.09,274.6ZM544,224a64,64,0,1,0-64-64A64.06,64.06,0,0,0,544,224ZM500.56,355.11a114.24,114.24,0,0,0-84.47-65.28L361,247.23c41.46-16.3,71-55.92,71-103.23A111.93,111.93,0,0,0,320,32c-57.14,0-103.69,42.83-110.6,98.08L45.46,3.38A16,16,0,0,0,23,6.19L3.37,31.46A16,16,0,0,0,6.18,53.91L594.53,508.63A16,16,0,0,0,617,505.82l19.64-25.27a16,16,0,0,0-2.81-22.45ZM128,403.21V432a48,48,0,0,0,48,48H464a47.45,47.45,0,0,0,12.57-1.87L232,289.13C173.74,294.83,128,343.42,128,403.21ZM576,256H512a63.79,63.79,0,0,0-45.09,18.6A146.29,146.29,0,0,1,542,384h66a32,32,0,0,0,32-32V320A64.06,64.06,0,0,0,576,256Z"></path></svg> `\(~~~\)`**Compared to the single-analyst condition** <svg viewBox="0 0 512 512" style="position:relative;display:inline-block;top:.1em;fill:#035AA6;height:1.5em;" xmlns="http://www.w3.org/2000/svg"> <path d="M504 255.531c.253 136.64-111.18 248.372-247.82 248.468-59.015.042-113.223-20.53-155.822-54.911-11.077-8.94-11.905-25.541-1.839-35.607l11.267-11.267c8.609-8.609 22.353-9.551 31.891-1.984C173.062 425.135 212.781 440 256 440c101.705 0 184-82.311 184-184 0-101.705-82.311-184-184-184-48.814 0-93.149 18.969-126.068 49.932l50.754 50.754c10.08 10.08 2.941 27.314-11.313 27.314H24c-8.837 0-16-7.163-16-16V38.627c0-14.254 17.234-21.393 27.314-11.314l49.372 49.372C129.209 34.136 189.552 8 256 8c136.81 0 247.747 110.78 248 247.531zm-180.912 78.784l9.823-12.63c8.138-10.463 6.253-25.542-4.21-33.679L288 256.349V152c0-13.255-10.745-24-24-24h-16c-13.255 0-24 10.745-24 24v135.651l65.409 50.874c10.463 8.137 25.541 6.253 33.679-4.21z"></path></svg> `\(~~~~\)`**Controlling for prior beliefs** <svg viewBox="0 0 512 512" style="position:relative;display:inline-block;top:.1em;fill:#035AA6;height:1.5em;" xmlns="http://www.w3.org/2000/svg"> <g groupmode="layer" id="layer6" label="icon"> <path id="path246" style="fill-opacity:1;fill-rule:nonzero;stroke:none;stroke-width:0.0352777" d="m 255.96701,7.9999992 c -14.42056,0 -28.9134,3.1667198 -39.84197,9.5105508 L 69.402174,102.20596 c -21.954611,12.67476 -39.899208,43.75438 -39.899208,69.08441 v 169.41924 c 0,25.33653 17.944597,56.42568 39.899208,69.0939 L 216.12504,494.51788 C 227.05361,500.84232 241.54645,504 255.96701,504 c 14.48556,0 28.97013,-3.15768 39.89917,-9.48212 l 146.7224,-84.71437 c 21.92213,-12.66822 39.90845,-43.75737 39.90845,-69.0939 V 171.29037 c 0,-25.33003 -17.98632,-56.40965 -39.90845,-69.08441 L 295.86618,17.51055 C 284.93714,11.166719 270.45257,7.9999992 255.96701,7.9999992 Z M 355.1558,152.37339 c 4.22726,0 8.45019,1.60138 11.65118,4.81215 l 17.84669,17.82761 c 6.402,6.42793 6.402,16.93826 0,23.3597 L 228.22149,354.82393 c -6.46654,6.41499 -16.93871,6.41499 -23.34074,0 l -68.94211,-68.91386 c -6.40202,-6.41497 -5.044,-18.2773 0,-23.34073 l 17.85586,-17.82761 c 6.40202,-6.42793 16.9387,-6.42793 23.34073,0 l 27.74552,27.72655 c 6.40203,6.415 16.8742,6.415 23.34074,0 L 343.45702,157.18554 c 3.23307,-3.21072 7.47102,-4.81215 11.69878,-4.81215 z"></path> </g></svg> `\(~~~\)` [**Preregistration at osf.io/rpu98**](https://osf.io/rpu98) ] .pull-right[ | Measure | Multi-consistent | Multi-inconsistent | | ------------- | ------------- | ------------- | | Posterior beliefs | .center[<svg viewBox="0 0 640 512" style="position:relative;display:inline-block;top:.1em;fill:green;height:1.5em;" xmlns="http://www.w3.org/2000/svg"> <path d="M624 208h-64v-64c0-8.8-7.2-16-16-16h-32c-8.8 0-16 7.2-16 16v64h-64c-8.8 0-16 7.2-16 16v32c0 8.8 7.2 16 16 16h64v64c0 8.8 7.2 16 16 16h32c8.8 0 16-7.2 16-16v-64h64c8.8 0 16-7.2 16-16v-32c0-8.8-7.2-16-16-16zm-400 48c70.7 0 128-57.3 128-128S294.7 0 224 0 96 57.3 96 128s57.3 128 128 128zm89.6 32h-16.7c-22.2 10.2-46.9 16-72.9 16s-50.6-5.8-72.9-16h-16.7C60.2 288 0 348.2 0 422.4V464c0 26.5 21.5 48 48 48h352c26.5 0 48-21.5 48-48v-41.6c0-74.2-60.2-134.4-134.4-134.4z"></path></svg>] | .center[<svg viewBox="0 0 640 512" style="position:relative;display:inline-block;top:.1em;fill:red;height:1.5em;" xmlns="http://www.w3.org/2000/svg"> <path d="M624 208H432c-8.8 0-16 7.2-16 16v32c0 8.8 7.2 16 16 16h192c8.8 0 16-7.2 16-16v-32c0-8.8-7.2-16-16-16zm-400 48c70.7 0 128-57.3 128-128S294.7 0 224 0 96 57.3 96 128s57.3 128 128 128zm89.6 32h-16.7c-22.2 10.2-46.9 16-72.9 16s-50.6-5.8-72.9-16h-16.7C60.2 288 0 348.2 0 422.4V464c0 26.5 21.5 48 48 48h352c26.5 0 48-21.5 48-48v-41.6c0-74.2-60.2-134.4-134.4-134.4z"></path></svg>] | |Credibility | .center[<svg viewBox="0 0 640 512" style="position:relative;display:inline-block;top:.1em;fill:green;height:1.5em;" xmlns="http://www.w3.org/2000/svg"> <path d="M624 208h-64v-64c0-8.8-7.2-16-16-16h-32c-8.8 0-16 7.2-16 16v64h-64c-8.8 0-16 7.2-16 16v32c0 8.8 7.2 16 16 16h64v64c0 8.8 7.2 16 16 16h32c8.8 0 16-7.2 16-16v-64h64c8.8 0 16-7.2 16-16v-32c0-8.8-7.2-16-16-16zm-400 48c70.7 0 128-57.3 128-128S294.7 0 224 0 96 57.3 96 128s57.3 128 128 128zm89.6 32h-16.7c-22.2 10.2-46.9 16-72.9 16s-50.6-5.8-72.9-16h-16.7C60.2 288 0 348.2 0 422.4V464c0 26.5 21.5 48 48 48h352c26.5 0 48-21.5 48-48v-41.6c0-74.2-60.2-134.4-134.4-134.4z"></path></svg>] | .center[<svg viewBox="0 0 640 512" style="position:relative;display:inline-block;top:.1em;fill:red;height:1.5em;" xmlns="http://www.w3.org/2000/svg"> <path d="M624 208H432c-8.8 0-16 7.2-16 16v32c0 8.8 7.2 16 16 16h192c8.8 0 16-7.2 16-16v-32c0-8.8-7.2-16-16-16zm-400 48c70.7 0 128-57.3 128-128S294.7 0 224 0 96 57.3 96 128s57.3 128 128 128zm89.6 32h-16.7c-22.2 10.2-46.9 16-72.9 16s-50.6-5.8-72.9-16h-16.7C60.2 288 0 348.2 0 422.4V464c0 26.5 21.5 48 48 48h352c26.5 0 48-21.5 48-48v-41.6c0-74.2-60.2-134.4-134.4-134.4z"></path></svg>] | ] ??? *Note*. Table indicates the predicted direction of the effect for all dependent variables, compared to the single-analyst condition and controlling for prior beliefs. For example, we hypothesized that, compared to a single-analyst study and controlling for prior beliefs, ratings of credibility would be greater in the multi-analyst: consistent condition and lower in the multi-analyst: inconsistent condition. Our pre-registered hypotheses can be found in the table: we hypothesized that in the multi-consistent condition (compared to the single-analyst condition), lay consumers would have higher posterior beliefs, would find the results more credible, and would be less likely to believe the results stem from bias or error. For the multi-inconsistent condition, we hypothesized that lay consumers would have lower posterior beliefs, would find the results less credible, and would be more likely to believe the results stem from bias or error. In addition, we expected that the act of providing multiple (slightly to widely varying) parameter estimates would decrease confidence in the aggregate parameter estimate in both multi-analyst conditions. --- .center[ # Hypotheses ] `\(~~\)` .pull-left[ <svg viewBox="0 0 496 512" style="position:relative;display:inline-block;top:.1em;fill:#035AA6;height:1.5em;" xmlns="http://www.w3.org/2000/svg"> <path d="M347.94 129.86L203.6 195.83a31.938 31.938 0 0 0-15.77 15.77l-65.97 144.34c-7.61 16.65 9.54 33.81 26.2 26.2l144.34-65.97a31.938 31.938 0 0 0 15.77-15.77l65.97-144.34c7.61-16.66-9.54-33.81-26.2-26.2zm-77.36 148.72c-12.47 12.47-32.69 12.47-45.16 0-12.47-12.47-12.47-32.69 0-45.16 12.47-12.47 32.69-12.47 45.16 0 12.47 12.47 12.47 32.69 0 45.16zM248 8C111.03 8 0 119.03 0 256s111.03 248 248 248 248-111.03 248-248S384.97 8 248 8zm0 448c-110.28 0-200-89.72-200-200S137.72 56 248 56s200 89.72 200 200-89.72 200-200 200z"></path></svg> `\(~~~~\)`**Predicted direction of effects** <svg viewBox="0 0 640 512" style="position:relative;display:inline-block;top:.1em;fill:#035AA6;height:1.5em;" xmlns="http://www.w3.org/2000/svg"> <path d="M132.65,212.32,36.21,137.78A63.4,63.4,0,0,0,32,160a63.84,63.84,0,0,0,100.65,52.32Zm40.44,62.28A63.79,63.79,0,0,0,128,256H64A64.06,64.06,0,0,0,0,320v32a32,32,0,0,0,32,32H97.91A146.62,146.62,0,0,1,173.09,274.6ZM544,224a64,64,0,1,0-64-64A64.06,64.06,0,0,0,544,224ZM500.56,355.11a114.24,114.24,0,0,0-84.47-65.28L361,247.23c41.46-16.3,71-55.92,71-103.23A111.93,111.93,0,0,0,320,32c-57.14,0-103.69,42.83-110.6,98.08L45.46,3.38A16,16,0,0,0,23,6.19L3.37,31.46A16,16,0,0,0,6.18,53.91L594.53,508.63A16,16,0,0,0,617,505.82l19.64-25.27a16,16,0,0,0-2.81-22.45ZM128,403.21V432a48,48,0,0,0,48,48H464a47.45,47.45,0,0,0,12.57-1.87L232,289.13C173.74,294.83,128,343.42,128,403.21ZM576,256H512a63.79,63.79,0,0,0-45.09,18.6A146.29,146.29,0,0,1,542,384h66a32,32,0,0,0,32-32V320A64.06,64.06,0,0,0,576,256Z"></path></svg> `\(~~~\)`**Compared to the single-analyst condition** <svg viewBox="0 0 512 512" style="position:relative;display:inline-block;top:.1em;fill:#035AA6;height:1.5em;" xmlns="http://www.w3.org/2000/svg"> <path d="M504 255.531c.253 136.64-111.18 248.372-247.82 248.468-59.015.042-113.223-20.53-155.822-54.911-11.077-8.94-11.905-25.541-1.839-35.607l11.267-11.267c8.609-8.609 22.353-9.551 31.891-1.984C173.062 425.135 212.781 440 256 440c101.705 0 184-82.311 184-184 0-101.705-82.311-184-184-184-48.814 0-93.149 18.969-126.068 49.932l50.754 50.754c10.08 10.08 2.941 27.314-11.313 27.314H24c-8.837 0-16-7.163-16-16V38.627c0-14.254 17.234-21.393 27.314-11.314l49.372 49.372C129.209 34.136 189.552 8 256 8c136.81 0 247.747 110.78 248 247.531zm-180.912 78.784l9.823-12.63c8.138-10.463 6.253-25.542-4.21-33.679L288 256.349V152c0-13.255-10.745-24-24-24h-16c-13.255 0-24 10.745-24 24v135.651l65.409 50.874c10.463 8.137 25.541 6.253 33.679-4.21z"></path></svg> `\(~~~~\)`**Controlling for prior beliefs** <svg viewBox="0 0 512 512" style="position:relative;display:inline-block;top:.1em;fill:#035AA6;height:1.5em;" xmlns="http://www.w3.org/2000/svg"> <g groupmode="layer" id="layer6" label="icon"> <path id="path246" style="fill-opacity:1;fill-rule:nonzero;stroke:none;stroke-width:0.0352777" d="m 255.96701,7.9999992 c -14.42056,0 -28.9134,3.1667198 -39.84197,9.5105508 L 69.402174,102.20596 c -21.954611,12.67476 -39.899208,43.75438 -39.899208,69.08441 v 169.41924 c 0,25.33653 17.944597,56.42568 39.899208,69.0939 L 216.12504,494.51788 C 227.05361,500.84232 241.54645,504 255.96701,504 c 14.48556,0 28.97013,-3.15768 39.89917,-9.48212 l 146.7224,-84.71437 c 21.92213,-12.66822 39.90845,-43.75737 39.90845,-69.0939 V 171.29037 c 0,-25.33003 -17.98632,-56.40965 -39.90845,-69.08441 L 295.86618,17.51055 C 284.93714,11.166719 270.45257,7.9999992 255.96701,7.9999992 Z M 355.1558,152.37339 c 4.22726,0 8.45019,1.60138 11.65118,4.81215 l 17.84669,17.82761 c 6.402,6.42793 6.402,16.93826 0,23.3597 L 228.22149,354.82393 c -6.46654,6.41499 -16.93871,6.41499 -23.34074,0 l -68.94211,-68.91386 c -6.40202,-6.41497 -5.044,-18.2773 0,-23.34073 l 17.85586,-17.82761 c 6.40202,-6.42793 16.9387,-6.42793 23.34073,0 l 27.74552,27.72655 c 6.40203,6.415 16.8742,6.415 23.34074,0 L 343.45702,157.18554 c 3.23307,-3.21072 7.47102,-4.81215 11.69878,-4.81215 z"></path> </g></svg> `\(~~~\)` [**Preregistration at osf.io/rpu98**](https://osf.io/rpu98) ] .pull-right[ | Measure | Multi-consistent | Multi-inconsistent | | ------------- | ------------- | ------------- | | Posterior beliefs | .center[<svg viewBox="0 0 640 512" style="position:relative;display:inline-block;top:.1em;fill:green;height:1.5em;" xmlns="http://www.w3.org/2000/svg"> <path d="M624 208h-64v-64c0-8.8-7.2-16-16-16h-32c-8.8 0-16 7.2-16 16v64h-64c-8.8 0-16 7.2-16 16v32c0 8.8 7.2 16 16 16h64v64c0 8.8 7.2 16 16 16h32c8.8 0 16-7.2 16-16v-64h64c8.8 0 16-7.2 16-16v-32c0-8.8-7.2-16-16-16zm-400 48c70.7 0 128-57.3 128-128S294.7 0 224 0 96 57.3 96 128s57.3 128 128 128zm89.6 32h-16.7c-22.2 10.2-46.9 16-72.9 16s-50.6-5.8-72.9-16h-16.7C60.2 288 0 348.2 0 422.4V464c0 26.5 21.5 48 48 48h352c26.5 0 48-21.5 48-48v-41.6c0-74.2-60.2-134.4-134.4-134.4z"></path></svg>] | .center[<svg viewBox="0 0 640 512" style="position:relative;display:inline-block;top:.1em;fill:red;height:1.5em;" xmlns="http://www.w3.org/2000/svg"> <path d="M624 208H432c-8.8 0-16 7.2-16 16v32c0 8.8 7.2 16 16 16h192c8.8 0 16-7.2 16-16v-32c0-8.8-7.2-16-16-16zm-400 48c70.7 0 128-57.3 128-128S294.7 0 224 0 96 57.3 96 128s57.3 128 128 128zm89.6 32h-16.7c-22.2 10.2-46.9 16-72.9 16s-50.6-5.8-72.9-16h-16.7C60.2 288 0 348.2 0 422.4V464c0 26.5 21.5 48 48 48h352c26.5 0 48-21.5 48-48v-41.6c0-74.2-60.2-134.4-134.4-134.4z"></path></svg>] | |Credibility | .center[<svg viewBox="0 0 640 512" style="position:relative;display:inline-block;top:.1em;fill:green;height:1.5em;" xmlns="http://www.w3.org/2000/svg"> <path d="M624 208h-64v-64c0-8.8-7.2-16-16-16h-32c-8.8 0-16 7.2-16 16v64h-64c-8.8 0-16 7.2-16 16v32c0 8.8 7.2 16 16 16h64v64c0 8.8 7.2 16 16 16h32c8.8 0 16-7.2 16-16v-64h64c8.8 0 16-7.2 16-16v-32c0-8.8-7.2-16-16-16zm-400 48c70.7 0 128-57.3 128-128S294.7 0 224 0 96 57.3 96 128s57.3 128 128 128zm89.6 32h-16.7c-22.2 10.2-46.9 16-72.9 16s-50.6-5.8-72.9-16h-16.7C60.2 288 0 348.2 0 422.4V464c0 26.5 21.5 48 48 48h352c26.5 0 48-21.5 48-48v-41.6c0-74.2-60.2-134.4-134.4-134.4z"></path></svg>] | .center[<svg viewBox="0 0 640 512" style="position:relative;display:inline-block;top:.1em;fill:red;height:1.5em;" xmlns="http://www.w3.org/2000/svg"> <path d="M624 208H432c-8.8 0-16 7.2-16 16v32c0 8.8 7.2 16 16 16h192c8.8 0 16-7.2 16-16v-32c0-8.8-7.2-16-16-16zm-400 48c70.7 0 128-57.3 128-128S294.7 0 224 0 96 57.3 96 128s57.3 128 128 128zm89.6 32h-16.7c-22.2 10.2-46.9 16-72.9 16s-50.6-5.8-72.9-16h-16.7C60.2 288 0 348.2 0 422.4V464c0 26.5 21.5 48 48 48h352c26.5 0 48-21.5 48-48v-41.6c0-74.2-60.2-134.4-134.4-134.4z"></path></svg>] | | Confidence | .center[<svg viewBox="0 0 640 512" style="position:relative;display:inline-block;top:.1em;fill:red;height:1.5em;" xmlns="http://www.w3.org/2000/svg"> <path d="M624 208H432c-8.8 0-16 7.2-16 16v32c0 8.8 7.2 16 16 16h192c8.8 0 16-7.2 16-16v-32c0-8.8-7.2-16-16-16zm-400 48c70.7 0 128-57.3 128-128S294.7 0 224 0 96 57.3 96 128s57.3 128 128 128zm89.6 32h-16.7c-22.2 10.2-46.9 16-72.9 16s-50.6-5.8-72.9-16h-16.7C60.2 288 0 348.2 0 422.4V464c0 26.5 21.5 48 48 48h352c26.5 0 48-21.5 48-48v-41.6c0-74.2-60.2-134.4-134.4-134.4z"></path></svg>] | .center[<svg viewBox="0 0 640 512" style="position:relative;display:inline-block;top:.1em;fill:red;height:1.5em;" xmlns="http://www.w3.org/2000/svg"> <path d="M624 208H432c-8.8 0-16 7.2-16 16v32c0 8.8 7.2 16 16 16h192c8.8 0 16-7.2 16-16v-32c0-8.8-7.2-16-16-16zm-400 48c70.7 0 128-57.3 128-128S294.7 0 224 0 96 57.3 96 128s57.3 128 128 128zm89.6 32h-16.7c-22.2 10.2-46.9 16-72.9 16s-50.6-5.8-72.9-16h-16.7C60.2 288 0 348.2 0 422.4V464c0 26.5 21.5 48 48 48h352c26.5 0 48-21.5 48-48v-41.6c0-74.2-60.2-134.4-134.4-134.4z"></path></svg>] | ] ??? *Note*. Table indicates the predicted direction of the effect for all dependent variables, compared to the single-analyst condition and controlling for prior beliefs. For example, we hypothesized that, compared to a single-analyst study and controlling for prior beliefs, ratings of credibility would be greater in the multi-analyst: consistent condition and lower in the multi-analyst: inconsistent condition. --- .center[ # Hypotheses ] `\(~~\)` .pull-left[ <svg viewBox="0 0 496 512" style="position:relative;display:inline-block;top:.1em;fill:#035AA6;height:1.5em;" xmlns="http://www.w3.org/2000/svg"> <path d="M347.94 129.86L203.6 195.83a31.938 31.938 0 0 0-15.77 15.77l-65.97 144.34c-7.61 16.65 9.54 33.81 26.2 26.2l144.34-65.97a31.938 31.938 0 0 0 15.77-15.77l65.97-144.34c7.61-16.66-9.54-33.81-26.2-26.2zm-77.36 148.72c-12.47 12.47-32.69 12.47-45.16 0-12.47-12.47-12.47-32.69 0-45.16 12.47-12.47 32.69-12.47 45.16 0 12.47 12.47 12.47 32.69 0 45.16zM248 8C111.03 8 0 119.03 0 256s111.03 248 248 248 248-111.03 248-248S384.97 8 248 8zm0 448c-110.28 0-200-89.72-200-200S137.72 56 248 56s200 89.72 200 200-89.72 200-200 200z"></path></svg> `\(~~~~\)`**Predicted direction of effects** <svg viewBox="0 0 640 512" style="position:relative;display:inline-block;top:.1em;fill:#035AA6;height:1.5em;" xmlns="http://www.w3.org/2000/svg"> <path d="M132.65,212.32,36.21,137.78A63.4,63.4,0,0,0,32,160a63.84,63.84,0,0,0,100.65,52.32Zm40.44,62.28A63.79,63.79,0,0,0,128,256H64A64.06,64.06,0,0,0,0,320v32a32,32,0,0,0,32,32H97.91A146.62,146.62,0,0,1,173.09,274.6ZM544,224a64,64,0,1,0-64-64A64.06,64.06,0,0,0,544,224ZM500.56,355.11a114.24,114.24,0,0,0-84.47-65.28L361,247.23c41.46-16.3,71-55.92,71-103.23A111.93,111.93,0,0,0,320,32c-57.14,0-103.69,42.83-110.6,98.08L45.46,3.38A16,16,0,0,0,23,6.19L3.37,31.46A16,16,0,0,0,6.18,53.91L594.53,508.63A16,16,0,0,0,617,505.82l19.64-25.27a16,16,0,0,0-2.81-22.45ZM128,403.21V432a48,48,0,0,0,48,48H464a47.45,47.45,0,0,0,12.57-1.87L232,289.13C173.74,294.83,128,343.42,128,403.21ZM576,256H512a63.79,63.79,0,0,0-45.09,18.6A146.29,146.29,0,0,1,542,384h66a32,32,0,0,0,32-32V320A64.06,64.06,0,0,0,576,256Z"></path></svg> `\(~~~\)`**Compared to the single-analyst condition** <svg viewBox="0 0 512 512" style="position:relative;display:inline-block;top:.1em;fill:#035AA6;height:1.5em;" xmlns="http://www.w3.org/2000/svg"> <path d="M504 255.531c.253 136.64-111.18 248.372-247.82 248.468-59.015.042-113.223-20.53-155.822-54.911-11.077-8.94-11.905-25.541-1.839-35.607l11.267-11.267c8.609-8.609 22.353-9.551 31.891-1.984C173.062 425.135 212.781 440 256 440c101.705 0 184-82.311 184-184 0-101.705-82.311-184-184-184-48.814 0-93.149 18.969-126.068 49.932l50.754 50.754c10.08 10.08 2.941 27.314-11.313 27.314H24c-8.837 0-16-7.163-16-16V38.627c0-14.254 17.234-21.393 27.314-11.314l49.372 49.372C129.209 34.136 189.552 8 256 8c136.81 0 247.747 110.78 248 247.531zm-180.912 78.784l9.823-12.63c8.138-10.463 6.253-25.542-4.21-33.679L288 256.349V152c0-13.255-10.745-24-24-24h-16c-13.255 0-24 10.745-24 24v135.651l65.409 50.874c10.463 8.137 25.541 6.253 33.679-4.21z"></path></svg> `\(~~~~\)`**Controlling for prior beliefs** <svg viewBox="0 0 512 512" style="position:relative;display:inline-block;top:.1em;fill:#035AA6;height:1.5em;" xmlns="http://www.w3.org/2000/svg"> <g groupmode="layer" id="layer6" label="icon"> <path id="path246" style="fill-opacity:1;fill-rule:nonzero;stroke:none;stroke-width:0.0352777" d="m 255.96701,7.9999992 c -14.42056,0 -28.9134,3.1667198 -39.84197,9.5105508 L 69.402174,102.20596 c -21.954611,12.67476 -39.899208,43.75438 -39.899208,69.08441 v 169.41924 c 0,25.33653 17.944597,56.42568 39.899208,69.0939 L 216.12504,494.51788 C 227.05361,500.84232 241.54645,504 255.96701,504 c 14.48556,0 28.97013,-3.15768 39.89917,-9.48212 l 146.7224,-84.71437 c 21.92213,-12.66822 39.90845,-43.75737 39.90845,-69.0939 V 171.29037 c 0,-25.33003 -17.98632,-56.40965 -39.90845,-69.08441 L 295.86618,17.51055 C 284.93714,11.166719 270.45257,7.9999992 255.96701,7.9999992 Z M 355.1558,152.37339 c 4.22726,0 8.45019,1.60138 11.65118,4.81215 l 17.84669,17.82761 c 6.402,6.42793 6.402,16.93826 0,23.3597 L 228.22149,354.82393 c -6.46654,6.41499 -16.93871,6.41499 -23.34074,0 l -68.94211,-68.91386 c -6.40202,-6.41497 -5.044,-18.2773 0,-23.34073 l 17.85586,-17.82761 c 6.40202,-6.42793 16.9387,-6.42793 23.34073,0 l 27.74552,27.72655 c 6.40203,6.415 16.8742,6.415 23.34074,0 L 343.45702,157.18554 c 3.23307,-3.21072 7.47102,-4.81215 11.69878,-4.81215 z"></path> </g></svg> `\(~~~\)` [**Preregistration at osf.io/rpu98**](https://osf.io/rpu98) ] .pull-right[ | Measure | Multi-consistent | Multi-inconsistent | | ------------- | ------------- | ------------- | | Posterior beliefs | .center[<svg viewBox="0 0 640 512" style="position:relative;display:inline-block;top:.1em;fill:green;height:1.5em;" xmlns="http://www.w3.org/2000/svg"> <path d="M624 208h-64v-64c0-8.8-7.2-16-16-16h-32c-8.8 0-16 7.2-16 16v64h-64c-8.8 0-16 7.2-16 16v32c0 8.8 7.2 16 16 16h64v64c0 8.8 7.2 16 16 16h32c8.8 0 16-7.2 16-16v-64h64c8.8 0 16-7.2 16-16v-32c0-8.8-7.2-16-16-16zm-400 48c70.7 0 128-57.3 128-128S294.7 0 224 0 96 57.3 96 128s57.3 128 128 128zm89.6 32h-16.7c-22.2 10.2-46.9 16-72.9 16s-50.6-5.8-72.9-16h-16.7C60.2 288 0 348.2 0 422.4V464c0 26.5 21.5 48 48 48h352c26.5 0 48-21.5 48-48v-41.6c0-74.2-60.2-134.4-134.4-134.4z"></path></svg>] | .center[<svg viewBox="0 0 640 512" style="position:relative;display:inline-block;top:.1em;fill:red;height:1.5em;" xmlns="http://www.w3.org/2000/svg"> <path d="M624 208H432c-8.8 0-16 7.2-16 16v32c0 8.8 7.2 16 16 16h192c8.8 0 16-7.2 16-16v-32c0-8.8-7.2-16-16-16zm-400 48c70.7 0 128-57.3 128-128S294.7 0 224 0 96 57.3 96 128s57.3 128 128 128zm89.6 32h-16.7c-22.2 10.2-46.9 16-72.9 16s-50.6-5.8-72.9-16h-16.7C60.2 288 0 348.2 0 422.4V464c0 26.5 21.5 48 48 48h352c26.5 0 48-21.5 48-48v-41.6c0-74.2-60.2-134.4-134.4-134.4z"></path></svg>] | |Credibility | .center[<svg viewBox="0 0 640 512" style="position:relative;display:inline-block;top:.1em;fill:green;height:1.5em;" xmlns="http://www.w3.org/2000/svg"> <path d="M624 208h-64v-64c0-8.8-7.2-16-16-16h-32c-8.8 0-16 7.2-16 16v64h-64c-8.8 0-16 7.2-16 16v32c0 8.8 7.2 16 16 16h64v64c0 8.8 7.2 16 16 16h32c8.8 0 16-7.2 16-16v-64h64c8.8 0 16-7.2 16-16v-32c0-8.8-7.2-16-16-16zm-400 48c70.7 0 128-57.3 128-128S294.7 0 224 0 96 57.3 96 128s57.3 128 128 128zm89.6 32h-16.7c-22.2 10.2-46.9 16-72.9 16s-50.6-5.8-72.9-16h-16.7C60.2 288 0 348.2 0 422.4V464c0 26.5 21.5 48 48 48h352c26.5 0 48-21.5 48-48v-41.6c0-74.2-60.2-134.4-134.4-134.4z"></path></svg>] | .center[<svg viewBox="0 0 640 512" style="position:relative;display:inline-block;top:.1em;fill:red;height:1.5em;" xmlns="http://www.w3.org/2000/svg"> <path d="M624 208H432c-8.8 0-16 7.2-16 16v32c0 8.8 7.2 16 16 16h192c8.8 0 16-7.2 16-16v-32c0-8.8-7.2-16-16-16zm-400 48c70.7 0 128-57.3 128-128S294.7 0 224 0 96 57.3 96 128s57.3 128 128 128zm89.6 32h-16.7c-22.2 10.2-46.9 16-72.9 16s-50.6-5.8-72.9-16h-16.7C60.2 288 0 348.2 0 422.4V464c0 26.5 21.5 48 48 48h352c26.5 0 48-21.5 48-48v-41.6c0-74.2-60.2-134.4-134.4-134.4z"></path></svg>] | | Confidence | .center[<svg viewBox="0 0 640 512" style="position:relative;display:inline-block;top:.1em;fill:red;height:1.5em;" xmlns="http://www.w3.org/2000/svg"> <path d="M624 208H432c-8.8 0-16 7.2-16 16v32c0 8.8 7.2 16 16 16h192c8.8 0 16-7.2 16-16v-32c0-8.8-7.2-16-16-16zm-400 48c70.7 0 128-57.3 128-128S294.7 0 224 0 96 57.3 96 128s57.3 128 128 128zm89.6 32h-16.7c-22.2 10.2-46.9 16-72.9 16s-50.6-5.8-72.9-16h-16.7C60.2 288 0 348.2 0 422.4V464c0 26.5 21.5 48 48 48h352c26.5 0 48-21.5 48-48v-41.6c0-74.2-60.2-134.4-134.4-134.4z"></path></svg>] | .center[<svg viewBox="0 0 640 512" style="position:relative;display:inline-block;top:.1em;fill:red;height:1.5em;" xmlns="http://www.w3.org/2000/svg"> <path d="M624 208H432c-8.8 0-16 7.2-16 16v32c0 8.8 7.2 16 16 16h192c8.8 0 16-7.2 16-16v-32c0-8.8-7.2-16-16-16zm-400 48c70.7 0 128-57.3 128-128S294.7 0 224 0 96 57.3 96 128s57.3 128 128 128zm89.6 32h-16.7c-22.2 10.2-46.9 16-72.9 16s-50.6-5.8-72.9-16h-16.7C60.2 288 0 348.2 0 422.4V464c0 26.5 21.5 48 48 48h352c26.5 0 48-21.5 48-48v-41.6c0-74.2-60.2-134.4-134.4-134.4z"></path></svg>] | | Bias | .center[<svg viewBox="0 0 640 512" style="position:relative;display:inline-block;top:.1em;fill:red;height:1.5em;" xmlns="http://www.w3.org/2000/svg"> <path d="M624 208H432c-8.8 0-16 7.2-16 16v32c0 8.8 7.2 16 16 16h192c8.8 0 16-7.2 16-16v-32c0-8.8-7.2-16-16-16zm-400 48c70.7 0 128-57.3 128-128S294.7 0 224 0 96 57.3 96 128s57.3 128 128 128zm89.6 32h-16.7c-22.2 10.2-46.9 16-72.9 16s-50.6-5.8-72.9-16h-16.7C60.2 288 0 348.2 0 422.4V464c0 26.5 21.5 48 48 48h352c26.5 0 48-21.5 48-48v-41.6c0-74.2-60.2-134.4-134.4-134.4z"></path></svg>] | .center[<svg viewBox="0 0 640 512" style="position:relative;display:inline-block;top:.1em;fill:green;height:1.5em;" xmlns="http://www.w3.org/2000/svg"> <path d="M624 208h-64v-64c0-8.8-7.2-16-16-16h-32c-8.8 0-16 7.2-16 16v64h-64c-8.8 0-16 7.2-16 16v32c0 8.8 7.2 16 16 16h64v64c0 8.8 7.2 16 16 16h32c8.8 0 16-7.2 16-16v-64h64c8.8 0 16-7.2 16-16v-32c0-8.8-7.2-16-16-16zm-400 48c70.7 0 128-57.3 128-128S294.7 0 224 0 96 57.3 96 128s57.3 128 128 128zm89.6 32h-16.7c-22.2 10.2-46.9 16-72.9 16s-50.6-5.8-72.9-16h-16.7C60.2 288 0 348.2 0 422.4V464c0 26.5 21.5 48 48 48h352c26.5 0 48-21.5 48-48v-41.6c0-74.2-60.2-134.4-134.4-134.4z"></path></svg>] | ] ??? *Note*. Table indicates the predicted direction of the effect for all dependent variables, compared to the single-analyst condition and controlling for prior beliefs. For example, we hypothesized that, compared to a single-analyst study and controlling for prior beliefs, ratings of credibility would be greater in the multi-analyst: consistent condition and lower in the multi-analyst: inconsistent condition. --- .center[ # Hypotheses ] `\(~~\)` .pull-left[ <svg viewBox="0 0 496 512" style="position:relative;display:inline-block;top:.1em;fill:#035AA6;height:1.5em;" xmlns="http://www.w3.org/2000/svg"> <path d="M347.94 129.86L203.6 195.83a31.938 31.938 0 0 0-15.77 15.77l-65.97 144.34c-7.61 16.65 9.54 33.81 26.2 26.2l144.34-65.97a31.938 31.938 0 0 0 15.77-15.77l65.97-144.34c7.61-16.66-9.54-33.81-26.2-26.2zm-77.36 148.72c-12.47 12.47-32.69 12.47-45.16 0-12.47-12.47-12.47-32.69 0-45.16 12.47-12.47 32.69-12.47 45.16 0 12.47 12.47 12.47 32.69 0 45.16zM248 8C111.03 8 0 119.03 0 256s111.03 248 248 248 248-111.03 248-248S384.97 8 248 8zm0 448c-110.28 0-200-89.72-200-200S137.72 56 248 56s200 89.72 200 200-89.72 200-200 200z"></path></svg> `\(~~~~\)`**Predicted direction of effects** <svg viewBox="0 0 640 512" style="position:relative;display:inline-block;top:.1em;fill:#035AA6;height:1.5em;" xmlns="http://www.w3.org/2000/svg"> <path d="M132.65,212.32,36.21,137.78A63.4,63.4,0,0,0,32,160a63.84,63.84,0,0,0,100.65,52.32Zm40.44,62.28A63.79,63.79,0,0,0,128,256H64A64.06,64.06,0,0,0,0,320v32a32,32,0,0,0,32,32H97.91A146.62,146.62,0,0,1,173.09,274.6ZM544,224a64,64,0,1,0-64-64A64.06,64.06,0,0,0,544,224ZM500.56,355.11a114.24,114.24,0,0,0-84.47-65.28L361,247.23c41.46-16.3,71-55.92,71-103.23A111.93,111.93,0,0,0,320,32c-57.14,0-103.69,42.83-110.6,98.08L45.46,3.38A16,16,0,0,0,23,6.19L3.37,31.46A16,16,0,0,0,6.18,53.91L594.53,508.63A16,16,0,0,0,617,505.82l19.64-25.27a16,16,0,0,0-2.81-22.45ZM128,403.21V432a48,48,0,0,0,48,48H464a47.45,47.45,0,0,0,12.57-1.87L232,289.13C173.74,294.83,128,343.42,128,403.21ZM576,256H512a63.79,63.79,0,0,0-45.09,18.6A146.29,146.29,0,0,1,542,384h66a32,32,0,0,0,32-32V320A64.06,64.06,0,0,0,576,256Z"></path></svg> `\(~~~\)`**Compared to the single-analyst condition** <svg viewBox="0 0 512 512" style="position:relative;display:inline-block;top:.1em;fill:#035AA6;height:1.5em;" xmlns="http://www.w3.org/2000/svg"> <path d="M504 255.531c.253 136.64-111.18 248.372-247.82 248.468-59.015.042-113.223-20.53-155.822-54.911-11.077-8.94-11.905-25.541-1.839-35.607l11.267-11.267c8.609-8.609 22.353-9.551 31.891-1.984C173.062 425.135 212.781 440 256 440c101.705 0 184-82.311 184-184 0-101.705-82.311-184-184-184-48.814 0-93.149 18.969-126.068 49.932l50.754 50.754c10.08 10.08 2.941 27.314-11.313 27.314H24c-8.837 0-16-7.163-16-16V38.627c0-14.254 17.234-21.393 27.314-11.314l49.372 49.372C129.209 34.136 189.552 8 256 8c136.81 0 247.747 110.78 248 247.531zm-180.912 78.784l9.823-12.63c8.138-10.463 6.253-25.542-4.21-33.679L288 256.349V152c0-13.255-10.745-24-24-24h-16c-13.255 0-24 10.745-24 24v135.651l65.409 50.874c10.463 8.137 25.541 6.253 33.679-4.21z"></path></svg> `\(~~~~\)`**Controlling for prior beliefs** <svg viewBox="0 0 512 512" style="position:relative;display:inline-block;top:.1em;fill:#035AA6;height:1.5em;" xmlns="http://www.w3.org/2000/svg"> <g groupmode="layer" id="layer6" label="icon"> <path id="path246" style="fill-opacity:1;fill-rule:nonzero;stroke:none;stroke-width:0.0352777" d="m 255.96701,7.9999992 c -14.42056,0 -28.9134,3.1667198 -39.84197,9.5105508 L 69.402174,102.20596 c -21.954611,12.67476 -39.899208,43.75438 -39.899208,69.08441 v 169.41924 c 0,25.33653 17.944597,56.42568 39.899208,69.0939 L 216.12504,494.51788 C 227.05361,500.84232 241.54645,504 255.96701,504 c 14.48556,0 28.97013,-3.15768 39.89917,-9.48212 l 146.7224,-84.71437 c 21.92213,-12.66822 39.90845,-43.75737 39.90845,-69.0939 V 171.29037 c 0,-25.33003 -17.98632,-56.40965 -39.90845,-69.08441 L 295.86618,17.51055 C 284.93714,11.166719 270.45257,7.9999992 255.96701,7.9999992 Z M 355.1558,152.37339 c 4.22726,0 8.45019,1.60138 11.65118,4.81215 l 17.84669,17.82761 c 6.402,6.42793 6.402,16.93826 0,23.3597 L 228.22149,354.82393 c -6.46654,6.41499 -16.93871,6.41499 -23.34074,0 l -68.94211,-68.91386 c -6.40202,-6.41497 -5.044,-18.2773 0,-23.34073 l 17.85586,-17.82761 c 6.40202,-6.42793 16.9387,-6.42793 23.34073,0 l 27.74552,27.72655 c 6.40203,6.415 16.8742,6.415 23.34074,0 L 343.45702,157.18554 c 3.23307,-3.21072 7.47102,-4.81215 11.69878,-4.81215 z"></path> </g></svg> `\(~~~\)` [**Preregistration at osf.io/rpu98**](https://osf.io/rpu98) ] .pull-right[ | Measure | Multi-consistent | Multi-inconsistent | | ------------- | ------------- | ------------- | | Posterior beliefs | .center[<svg viewBox="0 0 640 512" style="position:relative;display:inline-block;top:.1em;fill:green;height:1.5em;" xmlns="http://www.w3.org/2000/svg"> <path d="M624 208h-64v-64c0-8.8-7.2-16-16-16h-32c-8.8 0-16 7.2-16 16v64h-64c-8.8 0-16 7.2-16 16v32c0 8.8 7.2 16 16 16h64v64c0 8.8 7.2 16 16 16h32c8.8 0 16-7.2 16-16v-64h64c8.8 0 16-7.2 16-16v-32c0-8.8-7.2-16-16-16zm-400 48c70.7 0 128-57.3 128-128S294.7 0 224 0 96 57.3 96 128s57.3 128 128 128zm89.6 32h-16.7c-22.2 10.2-46.9 16-72.9 16s-50.6-5.8-72.9-16h-16.7C60.2 288 0 348.2 0 422.4V464c0 26.5 21.5 48 48 48h352c26.5 0 48-21.5 48-48v-41.6c0-74.2-60.2-134.4-134.4-134.4z"></path></svg>] | .center[<svg viewBox="0 0 640 512" style="position:relative;display:inline-block;top:.1em;fill:red;height:1.5em;" xmlns="http://www.w3.org/2000/svg"> <path d="M624 208H432c-8.8 0-16 7.2-16 16v32c0 8.8 7.2 16 16 16h192c8.8 0 16-7.2 16-16v-32c0-8.8-7.2-16-16-16zm-400 48c70.7 0 128-57.3 128-128S294.7 0 224 0 96 57.3 96 128s57.3 128 128 128zm89.6 32h-16.7c-22.2 10.2-46.9 16-72.9 16s-50.6-5.8-72.9-16h-16.7C60.2 288 0 348.2 0 422.4V464c0 26.5 21.5 48 48 48h352c26.5 0 48-21.5 48-48v-41.6c0-74.2-60.2-134.4-134.4-134.4z"></path></svg>] | |Credibility | .center[<svg viewBox="0 0 640 512" style="position:relative;display:inline-block;top:.1em;fill:green;height:1.5em;" xmlns="http://www.w3.org/2000/svg"> <path d="M624 208h-64v-64c0-8.8-7.2-16-16-16h-32c-8.8 0-16 7.2-16 16v64h-64c-8.8 0-16 7.2-16 16v32c0 8.8 7.2 16 16 16h64v64c0 8.8 7.2 16 16 16h32c8.8 0 16-7.2 16-16v-64h64c8.8 0 16-7.2 16-16v-32c0-8.8-7.2-16-16-16zm-400 48c70.7 0 128-57.3 128-128S294.7 0 224 0 96 57.3 96 128s57.3 128 128 128zm89.6 32h-16.7c-22.2 10.2-46.9 16-72.9 16s-50.6-5.8-72.9-16h-16.7C60.2 288 0 348.2 0 422.4V464c0 26.5 21.5 48 48 48h352c26.5 0 48-21.5 48-48v-41.6c0-74.2-60.2-134.4-134.4-134.4z"></path></svg>] | .center[<svg viewBox="0 0 640 512" style="position:relative;display:inline-block;top:.1em;fill:red;height:1.5em;" xmlns="http://www.w3.org/2000/svg"> <path d="M624 208H432c-8.8 0-16 7.2-16 16v32c0 8.8 7.2 16 16 16h192c8.8 0 16-7.2 16-16v-32c0-8.8-7.2-16-16-16zm-400 48c70.7 0 128-57.3 128-128S294.7 0 224 0 96 57.3 96 128s57.3 128 128 128zm89.6 32h-16.7c-22.2 10.2-46.9 16-72.9 16s-50.6-5.8-72.9-16h-16.7C60.2 288 0 348.2 0 422.4V464c0 26.5 21.5 48 48 48h352c26.5 0 48-21.5 48-48v-41.6c0-74.2-60.2-134.4-134.4-134.4z"></path></svg>] | | Confidence | .center[<svg viewBox="0 0 640 512" style="position:relative;display:inline-block;top:.1em;fill:red;height:1.5em;" xmlns="http://www.w3.org/2000/svg"> <path d="M624 208H432c-8.8 0-16 7.2-16 16v32c0 8.8 7.2 16 16 16h192c8.8 0 16-7.2 16-16v-32c0-8.8-7.2-16-16-16zm-400 48c70.7 0 128-57.3 128-128S294.7 0 224 0 96 57.3 96 128s57.3 128 128 128zm89.6 32h-16.7c-22.2 10.2-46.9 16-72.9 16s-50.6-5.8-72.9-16h-16.7C60.2 288 0 348.2 0 422.4V464c0 26.5 21.5 48 48 48h352c26.5 0 48-21.5 48-48v-41.6c0-74.2-60.2-134.4-134.4-134.4z"></path></svg>] | .center[<svg viewBox="0 0 640 512" style="position:relative;display:inline-block;top:.1em;fill:red;height:1.5em;" xmlns="http://www.w3.org/2000/svg"> <path d="M624 208H432c-8.8 0-16 7.2-16 16v32c0 8.8 7.2 16 16 16h192c8.8 0 16-7.2 16-16v-32c0-8.8-7.2-16-16-16zm-400 48c70.7 0 128-57.3 128-128S294.7 0 224 0 96 57.3 96 128s57.3 128 128 128zm89.6 32h-16.7c-22.2 10.2-46.9 16-72.9 16s-50.6-5.8-72.9-16h-16.7C60.2 288 0 348.2 0 422.4V464c0 26.5 21.5 48 48 48h352c26.5 0 48-21.5 48-48v-41.6c0-74.2-60.2-134.4-134.4-134.4z"></path></svg>] | | Bias | .center[<svg viewBox="0 0 640 512" style="position:relative;display:inline-block;top:.1em;fill:red;height:1.5em;" xmlns="http://www.w3.org/2000/svg"> <path d="M624 208H432c-8.8 0-16 7.2-16 16v32c0 8.8 7.2 16 16 16h192c8.8 0 16-7.2 16-16v-32c0-8.8-7.2-16-16-16zm-400 48c70.7 0 128-57.3 128-128S294.7 0 224 0 96 57.3 96 128s57.3 128 128 128zm89.6 32h-16.7c-22.2 10.2-46.9 16-72.9 16s-50.6-5.8-72.9-16h-16.7C60.2 288 0 348.2 0 422.4V464c0 26.5 21.5 48 48 48h352c26.5 0 48-21.5 48-48v-41.6c0-74.2-60.2-134.4-134.4-134.4z"></path></svg>] | .center[<svg viewBox="0 0 640 512" style="position:relative;display:inline-block;top:.1em;fill:green;height:1.5em;" xmlns="http://www.w3.org/2000/svg"> <path d="M624 208h-64v-64c0-8.8-7.2-16-16-16h-32c-8.8 0-16 7.2-16 16v64h-64c-8.8 0-16 7.2-16 16v32c0 8.8 7.2 16 16 16h64v64c0 8.8 7.2 16 16 16h32c8.8 0 16-7.2 16-16v-64h64c8.8 0 16-7.2 16-16v-32c0-8.8-7.2-16-16-16zm-400 48c70.7 0 128-57.3 128-128S294.7 0 224 0 96 57.3 96 128s57.3 128 128 128zm89.6 32h-16.7c-22.2 10.2-46.9 16-72.9 16s-50.6-5.8-72.9-16h-16.7C60.2 288 0 348.2 0 422.4V464c0 26.5 21.5 48 48 48h352c26.5 0 48-21.5 48-48v-41.6c0-74.2-60.2-134.4-134.4-134.4z"></path></svg>] | | Error | .center[<svg viewBox="0 0 640 512" style="position:relative;display:inline-block;top:.1em;fill:red;height:1.5em;" xmlns="http://www.w3.org/2000/svg"> <path d="M624 208H432c-8.8 0-16 7.2-16 16v32c0 8.8 7.2 16 16 16h192c8.8 0 16-7.2 16-16v-32c0-8.8-7.2-16-16-16zm-400 48c70.7 0 128-57.3 128-128S294.7 0 224 0 96 57.3 96 128s57.3 128 128 128zm89.6 32h-16.7c-22.2 10.2-46.9 16-72.9 16s-50.6-5.8-72.9-16h-16.7C60.2 288 0 348.2 0 422.4V464c0 26.5 21.5 48 48 48h352c26.5 0 48-21.5 48-48v-41.6c0-74.2-60.2-134.4-134.4-134.4z"></path></svg>] | .center[<svg viewBox="0 0 640 512" style="position:relative;display:inline-block;top:.1em;fill:green;height:1.5em;" xmlns="http://www.w3.org/2000/svg"> <path d="M624 208h-64v-64c0-8.8-7.2-16-16-16h-32c-8.8 0-16 7.2-16 16v64h-64c-8.8 0-16 7.2-16 16v32c0 8.8 7.2 16 16 16h64v64c0 8.8 7.2 16 16 16h32c8.8 0 16-7.2 16-16v-64h64c8.8 0 16-7.2 16-16v-32c0-8.8-7.2-16-16-16zm-400 48c70.7 0 128-57.3 128-128S294.7 0 224 0 96 57.3 96 128s57.3 128 128 128zm89.6 32h-16.7c-22.2 10.2-46.9 16-72.9 16s-50.6-5.8-72.9-16h-16.7C60.2 288 0 348.2 0 422.4V464c0 26.5 21.5 48 48 48h352c26.5 0 48-21.5 48-48v-41.6c0-74.2-60.2-134.4-134.4-134.4z"></path></svg>] | ] ??? *Note*. Table indicates the predicted direction of the effect for all dependent variables, compared to the single-analyst condition and controlling for prior beliefs. For example, we hypothesized that, compared to a single-analyst study and controlling for prior beliefs, ratings of credibility would be greater in the multi-analyst: consistent condition and lower in the multi-analyst: inconsistent condition. --- .center[ # Hypotheses ] `\(~~\)` .pull-left[ <svg viewBox="0 0 496 512" style="position:relative;display:inline-block;top:.1em;fill:#035AA6;height:1.5em;" xmlns="http://www.w3.org/2000/svg"> <path d="M347.94 129.86L203.6 195.83a31.938 31.938 0 0 0-15.77 15.77l-65.97 144.34c-7.61 16.65 9.54 33.81 26.2 26.2l144.34-65.97a31.938 31.938 0 0 0 15.77-15.77l65.97-144.34c7.61-16.66-9.54-33.81-26.2-26.2zm-77.36 148.72c-12.47 12.47-32.69 12.47-45.16 0-12.47-12.47-12.47-32.69 0-45.16 12.47-12.47 32.69-12.47 45.16 0 12.47 12.47 12.47 32.69 0 45.16zM248 8C111.03 8 0 119.03 0 256s111.03 248 248 248 248-111.03 248-248S384.97 8 248 8zm0 448c-110.28 0-200-89.72-200-200S137.72 56 248 56s200 89.72 200 200-89.72 200-200 200z"></path></svg> `\(~~~~\)`**Predicted direction of effects** <svg viewBox="0 0 640 512" style="position:relative;display:inline-block;top:.1em;fill:#035AA6;height:1.5em;" xmlns="http://www.w3.org/2000/svg"> <path d="M132.65,212.32,36.21,137.78A63.4,63.4,0,0,0,32,160a63.84,63.84,0,0,0,100.65,52.32Zm40.44,62.28A63.79,63.79,0,0,0,128,256H64A64.06,64.06,0,0,0,0,320v32a32,32,0,0,0,32,32H97.91A146.62,146.62,0,0,1,173.09,274.6ZM544,224a64,64,0,1,0-64-64A64.06,64.06,0,0,0,544,224ZM500.56,355.11a114.24,114.24,0,0,0-84.47-65.28L361,247.23c41.46-16.3,71-55.92,71-103.23A111.93,111.93,0,0,0,320,32c-57.14,0-103.69,42.83-110.6,98.08L45.46,3.38A16,16,0,0,0,23,6.19L3.37,31.46A16,16,0,0,0,6.18,53.91L594.53,508.63A16,16,0,0,0,617,505.82l19.64-25.27a16,16,0,0,0-2.81-22.45ZM128,403.21V432a48,48,0,0,0,48,48H464a47.45,47.45,0,0,0,12.57-1.87L232,289.13C173.74,294.83,128,343.42,128,403.21ZM576,256H512a63.79,63.79,0,0,0-45.09,18.6A146.29,146.29,0,0,1,542,384h66a32,32,0,0,0,32-32V320A64.06,64.06,0,0,0,576,256Z"></path></svg> `\(~~~\)`**Compared to the single-analyst condition** <svg viewBox="0 0 512 512" style="position:relative;display:inline-block;top:.1em;fill:#035AA6;height:1.5em;" xmlns="http://www.w3.org/2000/svg"> <path d="M504 255.531c.253 136.64-111.18 248.372-247.82 248.468-59.015.042-113.223-20.53-155.822-54.911-11.077-8.94-11.905-25.541-1.839-35.607l11.267-11.267c8.609-8.609 22.353-9.551 31.891-1.984C173.062 425.135 212.781 440 256 440c101.705 0 184-82.311 184-184 0-101.705-82.311-184-184-184-48.814 0-93.149 18.969-126.068 49.932l50.754 50.754c10.08 10.08 2.941 27.314-11.313 27.314H24c-8.837 0-16-7.163-16-16V38.627c0-14.254 17.234-21.393 27.314-11.314l49.372 49.372C129.209 34.136 189.552 8 256 8c136.81 0 247.747 110.78 248 247.531zm-180.912 78.784l9.823-12.63c8.138-10.463 6.253-25.542-4.21-33.679L288 256.349V152c0-13.255-10.745-24-24-24h-16c-13.255 0-24 10.745-24 24v135.651l65.409 50.874c10.463 8.137 25.541 6.253 33.679-4.21z"></path></svg> `\(~~~~\)`**Controlling for prior beliefs** <svg viewBox="0 0 512 512" style="position:relative;display:inline-block;top:.1em;fill:#035AA6;height:1.5em;" xmlns="http://www.w3.org/2000/svg"> <g groupmode="layer" id="layer6" label="icon"> <path id="path246" style="fill-opacity:1;fill-rule:nonzero;stroke:none;stroke-width:0.0352777" d="m 255.96701,7.9999992 c -14.42056,0 -28.9134,3.1667198 -39.84197,9.5105508 L 69.402174,102.20596 c -21.954611,12.67476 -39.899208,43.75438 -39.899208,69.08441 v 169.41924 c 0,25.33653 17.944597,56.42568 39.899208,69.0939 L 216.12504,494.51788 C 227.05361,500.84232 241.54645,504 255.96701,504 c 14.48556,0 28.97013,-3.15768 39.89917,-9.48212 l 146.7224,-84.71437 c 21.92213,-12.66822 39.90845,-43.75737 39.90845,-69.0939 V 171.29037 c 0,-25.33003 -17.98632,-56.40965 -39.90845,-69.08441 L 295.86618,17.51055 C 284.93714,11.166719 270.45257,7.9999992 255.96701,7.9999992 Z M 355.1558,152.37339 c 4.22726,0 8.45019,1.60138 11.65118,4.81215 l 17.84669,17.82761 c 6.402,6.42793 6.402,16.93826 0,23.3597 L 228.22149,354.82393 c -6.46654,6.41499 -16.93871,6.41499 -23.34074,0 l -68.94211,-68.91386 c -6.40202,-6.41497 -5.044,-18.2773 0,-23.34073 l 17.85586,-17.82761 c 6.40202,-6.42793 16.9387,-6.42793 23.34073,0 l 27.74552,27.72655 c 6.40203,6.415 16.8742,6.415 23.34074,0 L 343.45702,157.18554 c 3.23307,-3.21072 7.47102,-4.81215 11.69878,-4.81215 z"></path> </g></svg> `\(~~~\)` [**Preregistration at osf.io/rpu98**](https://osf.io/rpu98) ] .pull-right[ | Measure | Multi-consistent | Multi-inconsistent | | ------------- | ------------- | ------------- | | Posterior beliefs | .center[<svg viewBox="0 0 640 512" style="position:relative;display:inline-block;top:.1em;fill:green;height:1.5em;" xmlns="http://www.w3.org/2000/svg"> <path d="M624 208h-64v-64c0-8.8-7.2-16-16-16h-32c-8.8 0-16 7.2-16 16v64h-64c-8.8 0-16 7.2-16 16v32c0 8.8 7.2 16 16 16h64v64c0 8.8 7.2 16 16 16h32c8.8 0 16-7.2 16-16v-64h64c8.8 0 16-7.2 16-16v-32c0-8.8-7.2-16-16-16zm-400 48c70.7 0 128-57.3 128-128S294.7 0 224 0 96 57.3 96 128s57.3 128 128 128zm89.6 32h-16.7c-22.2 10.2-46.9 16-72.9 16s-50.6-5.8-72.9-16h-16.7C60.2 288 0 348.2 0 422.4V464c0 26.5 21.5 48 48 48h352c26.5 0 48-21.5 48-48v-41.6c0-74.2-60.2-134.4-134.4-134.4z"></path></svg>] | .center[<svg viewBox="0 0 640 512" style="position:relative;display:inline-block;top:.1em;fill:red;height:1.5em;" xmlns="http://www.w3.org/2000/svg"> <path d="M624 208H432c-8.8 0-16 7.2-16 16v32c0 8.8 7.2 16 16 16h192c8.8 0 16-7.2 16-16v-32c0-8.8-7.2-16-16-16zm-400 48c70.7 0 128-57.3 128-128S294.7 0 224 0 96 57.3 96 128s57.3 128 128 128zm89.6 32h-16.7c-22.2 10.2-46.9 16-72.9 16s-50.6-5.8-72.9-16h-16.7C60.2 288 0 348.2 0 422.4V464c0 26.5 21.5 48 48 48h352c26.5 0 48-21.5 48-48v-41.6c0-74.2-60.2-134.4-134.4-134.4z"></path></svg>] | |Credibility | .center[<svg viewBox="0 0 640 512" style="position:relative;display:inline-block;top:.1em;fill:green;height:1.5em;" xmlns="http://www.w3.org/2000/svg"> <path d="M624 208h-64v-64c0-8.8-7.2-16-16-16h-32c-8.8 0-16 7.2-16 16v64h-64c-8.8 0-16 7.2-16 16v32c0 8.8 7.2 16 16 16h64v64c0 8.8 7.2 16 16 16h32c8.8 0 16-7.2 16-16v-64h64c8.8 0 16-7.2 16-16v-32c0-8.8-7.2-16-16-16zm-400 48c70.7 0 128-57.3 128-128S294.7 0 224 0 96 57.3 96 128s57.3 128 128 128zm89.6 32h-16.7c-22.2 10.2-46.9 16-72.9 16s-50.6-5.8-72.9-16h-16.7C60.2 288 0 348.2 0 422.4V464c0 26.5 21.5 48 48 48h352c26.5 0 48-21.5 48-48v-41.6c0-74.2-60.2-134.4-134.4-134.4z"></path></svg>] | .center[<svg viewBox="0 0 640 512" style="position:relative;display:inline-block;top:.1em;fill:red;height:1.5em;" xmlns="http://www.w3.org/2000/svg"> <path d="M624 208H432c-8.8 0-16 7.2-16 16v32c0 8.8 7.2 16 16 16h192c8.8 0 16-7.2 16-16v-32c0-8.8-7.2-16-16-16zm-400 48c70.7 0 128-57.3 128-128S294.7 0 224 0 96 57.3 96 128s57.3 128 128 128zm89.6 32h-16.7c-22.2 10.2-46.9 16-72.9 16s-50.6-5.8-72.9-16h-16.7C60.2 288 0 348.2 0 422.4V464c0 26.5 21.5 48 48 48h352c26.5 0 48-21.5 48-48v-41.6c0-74.2-60.2-134.4-134.4-134.4z"></path></svg>] | | Confidence | .center[<svg viewBox="0 0 640 512" style="position:relative;display:inline-block;top:.1em;fill:red;height:1.5em;" xmlns="http://www.w3.org/2000/svg"> <path d="M624 208H432c-8.8 0-16 7.2-16 16v32c0 8.8 7.2 16 16 16h192c8.8 0 16-7.2 16-16v-32c0-8.8-7.2-16-16-16zm-400 48c70.7 0 128-57.3 128-128S294.7 0 224 0 96 57.3 96 128s57.3 128 128 128zm89.6 32h-16.7c-22.2 10.2-46.9 16-72.9 16s-50.6-5.8-72.9-16h-16.7C60.2 288 0 348.2 0 422.4V464c0 26.5 21.5 48 48 48h352c26.5 0 48-21.5 48-48v-41.6c0-74.2-60.2-134.4-134.4-134.4z"></path></svg>] | .center[<svg viewBox="0 0 640 512" style="position:relative;display:inline-block;top:.1em;fill:red;height:1.5em;" xmlns="http://www.w3.org/2000/svg"> <path d="M624 208H432c-8.8 0-16 7.2-16 16v32c0 8.8 7.2 16 16 16h192c8.8 0 16-7.2 16-16v-32c0-8.8-7.2-16-16-16zm-400 48c70.7 0 128-57.3 128-128S294.7 0 224 0 96 57.3 96 128s57.3 128 128 128zm89.6 32h-16.7c-22.2 10.2-46.9 16-72.9 16s-50.6-5.8-72.9-16h-16.7C60.2 288 0 348.2 0 422.4V464c0 26.5 21.5 48 48 48h352c26.5 0 48-21.5 48-48v-41.6c0-74.2-60.2-134.4-134.4-134.4z"></path></svg>] | | Bias | .center[<svg viewBox="0 0 640 512" style="position:relative;display:inline-block;top:.1em;fill:red;height:1.5em;" xmlns="http://www.w3.org/2000/svg"> <path d="M624 208H432c-8.8 0-16 7.2-16 16v32c0 8.8 7.2 16 16 16h192c8.8 0 16-7.2 16-16v-32c0-8.8-7.2-16-16-16zm-400 48c70.7 0 128-57.3 128-128S294.7 0 224 0 96 57.3 96 128s57.3 128 128 128zm89.6 32h-16.7c-22.2 10.2-46.9 16-72.9 16s-50.6-5.8-72.9-16h-16.7C60.2 288 0 348.2 0 422.4V464c0 26.5 21.5 48 48 48h352c26.5 0 48-21.5 48-48v-41.6c0-74.2-60.2-134.4-134.4-134.4z"></path></svg>] | .center[<svg viewBox="0 0 640 512" style="position:relative;display:inline-block;top:.1em;fill:green;height:1.5em;" xmlns="http://www.w3.org/2000/svg"> <path d="M624 208h-64v-64c0-8.8-7.2-16-16-16h-32c-8.8 0-16 7.2-16 16v64h-64c-8.8 0-16 7.2-16 16v32c0 8.8 7.2 16 16 16h64v64c0 8.8 7.2 16 16 16h32c8.8 0 16-7.2 16-16v-64h64c8.8 0 16-7.2 16-16v-32c0-8.8-7.2-16-16-16zm-400 48c70.7 0 128-57.3 128-128S294.7 0 224 0 96 57.3 96 128s57.3 128 128 128zm89.6 32h-16.7c-22.2 10.2-46.9 16-72.9 16s-50.6-5.8-72.9-16h-16.7C60.2 288 0 348.2 0 422.4V464c0 26.5 21.5 48 48 48h352c26.5 0 48-21.5 48-48v-41.6c0-74.2-60.2-134.4-134.4-134.4z"></path></svg>] | | Error | .center[<svg viewBox="0 0 640 512" style="position:relative;display:inline-block;top:.1em;fill:red;height:1.5em;" xmlns="http://www.w3.org/2000/svg"> <path d="M624 208H432c-8.8 0-16 7.2-16 16v32c0 8.8 7.2 16 16 16h192c8.8 0 16-7.2 16-16v-32c0-8.8-7.2-16-16-16zm-400 48c70.7 0 128-57.3 128-128S294.7 0 224 0 96 57.3 96 128s57.3 128 128 128zm89.6 32h-16.7c-22.2 10.2-46.9 16-72.9 16s-50.6-5.8-72.9-16h-16.7C60.2 288 0 348.2 0 422.4V464c0 26.5 21.5 48 48 48h352c26.5 0 48-21.5 48-48v-41.6c0-74.2-60.2-134.4-134.4-134.4z"></path></svg>] | .center[<svg viewBox="0 0 640 512" style="position:relative;display:inline-block;top:.1em;fill:green;height:1.5em;" xmlns="http://www.w3.org/2000/svg"> <path d="M624 208h-64v-64c0-8.8-7.2-16-16-16h-32c-8.8 0-16 7.2-16 16v64h-64c-8.8 0-16 7.2-16 16v32c0 8.8 7.2 16 16 16h64v64c0 8.8 7.2 16 16 16h32c8.8 0 16-7.2 16-16v-64h64c8.8 0 16-7.2 16-16v-32c0-8.8-7.2-16-16-16zm-400 48c70.7 0 128-57.3 128-128S294.7 0 224 0 96 57.3 96 128s57.3 128 128 128zm89.6 32h-16.7c-22.2 10.2-46.9 16-72.9 16s-50.6-5.8-72.9-16h-16.7C60.2 288 0 348.2 0 422.4V464c0 26.5 21.5 48 48 48h352c26.5 0 48-21.5 48-48v-41.6c0-74.2-60.2-134.4-134.4-134.4z"></path></svg>] | | Discretion | .center[No prediction] | .center[No prediction] | ] ??? *Note*. Table indicates the predicted direction of the effect for all dependent variables, compared to the single-analyst condition and controlling for prior beliefs. For example, we hypothesized that, compared to a single-analyst study and controlling for prior beliefs, ratings of credibility would be greater in the multi-analyst: consistent condition and lower in the multi-analyst: inconsistent condition. --- class: inverse, center, middle # Survey Materials --- .panelset.sideways[ .panel[.panel-name[Introduction] <img src="talk/introduction.png" width="90%" /> ] .panel[.panel-name[Prior Beliefs] 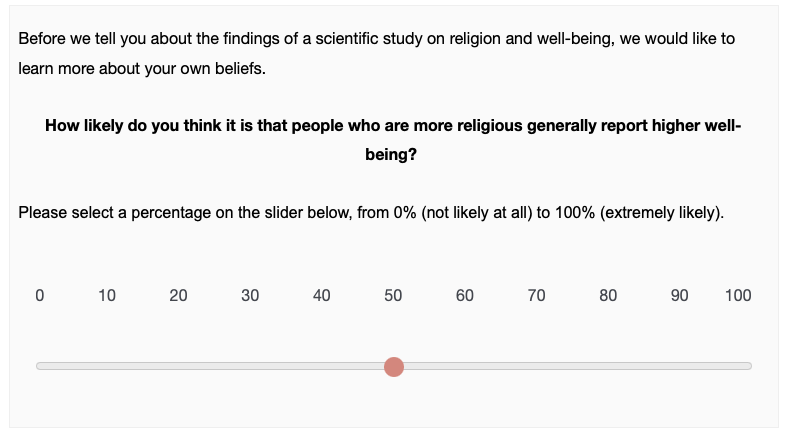 ] .panel[.panel-name[Thanks!] 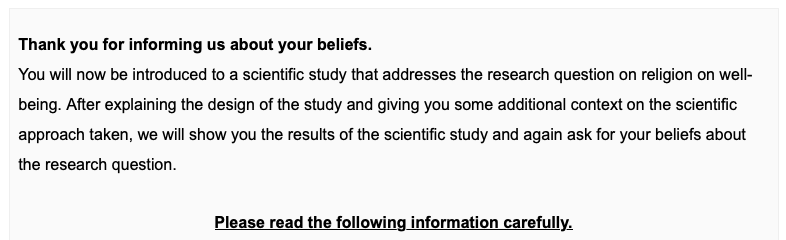 ] ] --- .panelset.sideways[ .panel[.panel-name[Study design & research approach (Single-analyst)] <img src="talk/design_single.png" width="93%" /> ] .panel[.panel-name[Study design & research approach (Multi-analyst)] <img src="talk/design_multi.png" width="91%" /> ] ] ??? Participants are first introduced to the research question and research approach. In the single-analyst condition, a single team of six researchers analyzes the same dataset, and in the two multi-analyst conditions, six independent researchers analyze the same dataset. Importantly, in all conditions we emphasize in the last paragraph that there are a lot of choices to be made. Participants had to wait 30 seconds before proceeding to the next slide. --- .panelset[ .panel[.panel-name[Results (Single-analyst)] <img src="talk/results_single.png" width="80%" /> ] .panel[.panel-name[Results (Multi-consistent)] <img src="talk/results_multi-consistent.png" width="80%" /> ] .panel[.panel-name[Results (Multi-inconsistent)] <img src="talk/results_multi-inconsistent.png" width="80%" /> ] ] ??? Here are the results we show participants. In the single-analyst condition, the team of six researchers concludes that religious people do indeed report higher well-being, and they find a 5% increase in well-being for every one point increase in religiosity. In the multi-consistent condition, the six independent researchers show consensus in that they all report a positive relationship, and the spread in their estimates is quite small; they range from 2% to 8%. In the multi-inconsistent condition, the six independent researchers do not show consensus, in that two of the scientists conclude that the relationship is negative, and four of the scientists conclude that the relationship is positive, and we can see that the spread in their estimates is quite large, ranging from -6% to 16%. --- .panelset.sideways[ .panel[.panel-name[Posterior beliefs] <img src="talk/posterior_beliefs.png" width="100%" /> ] .panel[.panel-name[Credibility] <img src="talk/credibility.png" width="100%" /> ] .panel[.panel-name[Confidence] <img src="talk/confidence.png" width="100%" /> ] .panel[.panel-name[Bias] <img src="talk/bias.png" width="100%" /> ] .panel[.panel-name[Error] <img src="talk/bias.png" width="100%" /> ] .panel[.panel-name[Discretion] <img src="talk/bias.png" width="100%" /> ] .panel[.panel-name[Attention check] <img src="talk/attention.png" width="100%" /> ] ] --- class: inverse, center, middle name: results # Results --- class: left # Participants -- <img src="talk/participants.png" width="110%" style="display: block; margin: auto;" /> ??? 500 in the multi-consistent condition, 499 in the single-analyst and the multi-inconsistent condition --- class: inverse, center, middle # Main results --- background-image: url("talk/Figure1b.jpg") background-position: 50% 50% background-size: 78% ??? For the results, we regressed all outcomes on prior beliefs and condition, with the single-analyst condition as the reference category. So all results that I will show you control for prior beliefs and show the results for the two multi-analyst conditions compared to the single-analyst condition. I will now walk you through each individual aspect of this Figure. --- background-image: url("talk/Figure1.jpg") background-position: 95% 57% background-size: 40% .center[ # Results: Multi-inconsistent ] **In line with our hypotheses**, lay consumers of multi-analyst studies with **inconsistent** results -- <svg viewBox="0 0 512 512" style="position:relative;display:inline-block;top:.1em;fill:red;height:1.5em;" xmlns="http://www.w3.org/2000/svg"> <path d="M504 256c0 137-111 248-248 248S8 393 8 256 119 8 256 8s248 111 248 248zm-143.6-28.9L288 302.6V120c0-13.3-10.7-24-24-24h-16c-13.3 0-24 10.7-24 24v182.6l-72.4-75.5c-9.3-9.7-24.8-9.9-34.3-.4l-10.9 11c-9.4 9.4-9.4 24.6 0 33.9L239 404.3c9.4 9.4 24.6 9.4 33.9 0l132.7-132.7c9.4-9.4 9.4-24.6 0-33.9l-10.9-11c-9.5-9.5-25-9.3-34.3.4z"></path></svg> `\(~~~\)` Have lower posterior beliefs -- <svg viewBox="0 0 512 512" style="position:relative;display:inline-block;top:.1em;fill:red;height:1.5em;" xmlns="http://www.w3.org/2000/svg"> <path d="M504 256c0 137-111 248-248 248S8 393 8 256 119 8 256 8s248 111 248 248zm-143.6-28.9L288 302.6V120c0-13.3-10.7-24-24-24h-16c-13.3 0-24 10.7-24 24v182.6l-72.4-75.5c-9.3-9.7-24.8-9.9-34.3-.4l-10.9 11c-9.4 9.4-9.4 24.6 0 33.9L239 404.3c9.4 9.4 24.6 9.4 33.9 0l132.7-132.7c9.4-9.4 9.4-24.6 0-33.9l-10.9-11c-9.5-9.5-25-9.3-34.3.4z"></path></svg> `\(~~~\)` Find the results less credible -- <svg viewBox="0 0 512 512" style="position:relative;display:inline-block;top:.1em;fill:red;height:1.5em;" xmlns="http://www.w3.org/2000/svg"> <path d="M504 256c0 137-111 248-248 248S8 393 8 256 119 8 256 8s248 111 248 248zm-143.6-28.9L288 302.6V120c0-13.3-10.7-24-24-24h-16c-13.3 0-24 10.7-24 24v182.6l-72.4-75.5c-9.3-9.7-24.8-9.9-34.3-.4l-10.9 11c-9.4 9.4-9.4 24.6 0 33.9L239 404.3c9.4 9.4 24.6 9.4 33.9 0l132.7-132.7c9.4-9.4 9.4-24.6 0-33.9l-10.9-11c-9.5-9.5-25-9.3-34.3.4z"></path></svg> `\(~~~\)` Have less confidence in the aggregate estimate of 5% -- <svg viewBox="0 0 512 512" style="position:relative;display:inline-block;top:.1em;fill:green;height:1.5em;" xmlns="http://www.w3.org/2000/svg"> <path d="M8 256C8 119 119 8 256 8s248 111 248 248-111 248-248 248S8 393 8 256zm143.6 28.9l72.4-75.5V392c0 13.3 10.7 24 24 24h16c13.3 0 24-10.7 24-24V209.4l72.4 75.5c9.3 9.7 24.8 9.9 34.3.4l10.9-11c9.4-9.4 9.4-24.6 0-33.9L273 107.7c-9.4-9.4-24.6-9.4-33.9 0L106.3 240.4c-9.4 9.4-9.4 24.6 0 33.9l10.9 11c9.6 9.5 25.1 9.3 34.4-.4z"></path></svg> `\(~~~\)` Believe the 5% estimate is more likely to stem from bias -- <svg viewBox="0 0 512 512" style="position:relative;display:inline-block;top:.1em;fill:green;height:1.5em;" xmlns="http://www.w3.org/2000/svg"> <path d="M8 256C8 119 119 8 256 8s248 111 248 248-111 248-248 248S8 393 8 256zm143.6 28.9l72.4-75.5V392c0 13.3 10.7 24 24 24h16c13.3 0 24-10.7 24-24V209.4l72.4 75.5c9.3 9.7 24.8 9.9 34.3.4l10.9-11c9.4-9.4 9.4-24.6 0-33.9L273 107.7c-9.4-9.4-24.6-9.4-33.9 0L106.3 240.4c-9.4 9.4-9.4 24.6 0 33.9l10.9 11c9.6 9.5 25.1 9.3 34.4-.4z"></path></svg> `\(~~~\)` Believe the 5% estimate is more likely to stem from error -- `\(~~\)` `\(~~\)` For the additional **exploratory measure**, lay consumers of multi-analyst studies with **inconsistent** results <svg viewBox="0 0 512 512" style="position:relative;display:inline-block;top:.1em;fill:green;height:1.5em;" xmlns="http://www.w3.org/2000/svg"> <path d="M8 256C8 119 119 8 256 8s248 111 248 248-111 248-248 248S8 393 8 256zm143.6 28.9l72.4-75.5V392c0 13.3 10.7 24 24 24h16c13.3 0 24-10.7 24-24V209.4l72.4 75.5c9.3 9.7 24.8 9.9 34.3.4l10.9-11c9.4-9.4 9.4-24.6 0-33.9L273 107.7c-9.4-9.4-24.6-9.4-33.9 0L106.3 240.4c-9.4 9.4-9.4 24.6 0 33.9l10.9 11c9.6 9.5 25.1 9.3 34.4-.4z"></path></svg> `\(~~~\)` Believe the 5% estimate is more likely to stem from idiosyncratic choices ??? ??? In line with out hypotheses, we find that lay consumers of multi-analyst studies with inconsistent results have lower posterior beliefs (you can see this estimate here), find the results less credible, have less confidence in the aggregate estimate of 5%, and believe this estimate is more likely to stem from bias, error, and discretion (which we define as idiosyncratic choices among multiple valid approaches, or what we researchers often refer to as researcher degrees of freedom). --- background-image: url("talk/Figure1.jpg") background-position: 95% 57% background-size: 40% .center[ # Results: Multi-consistent ] **Contrary to our hypotheses**, lay consumers of multi-analyst studies with **consistent** results -- <svg viewBox="0 0 512 512" style="position:relative;display:inline-block;top:.1em;fill:red;height:1.5em;" xmlns="http://www.w3.org/2000/svg"> <path d="M504 256c0 137-111 248-248 248S8 393 8 256 119 8 256 8s248 111 248 248zm-143.6-28.9L288 302.6V120c0-13.3-10.7-24-24-24h-16c-13.3 0-24 10.7-24 24v182.6l-72.4-75.5c-9.3-9.7-24.8-9.9-34.3-.4l-10.9 11c-9.4 9.4-9.4 24.6 0 33.9L239 404.3c9.4 9.4 24.6 9.4 33.9 0l132.7-132.7c9.4-9.4 9.4-24.6 0-33.9l-10.9-11c-9.5-9.5-25-9.3-34.3.4z"></path></svg> `\(~~~\)` Have lower posterior beliefs -- <svg viewBox="0 0 512 512" style="position:relative;display:inline-block;top:.1em;fill:green;height:1.5em;" xmlns="http://www.w3.org/2000/svg"> <path d="M8 256C8 119 119 8 256 8s248 111 248 248-111 248-248 248S8 393 8 256zm143.6 28.9l72.4-75.5V392c0 13.3 10.7 24 24 24h16c13.3 0 24-10.7 24-24V209.4l72.4 75.5c9.3 9.7 24.8 9.9 34.3.4l10.9-11c9.4-9.4 9.4-24.6 0-33.9L273 107.7c-9.4-9.4-24.6-9.4-33.9 0L106.3 240.4c-9.4 9.4-9.4 24.6 0 33.9l10.9 11c9.6 9.5 25.1 9.3 34.4-.4z"></path></svg> `\(~~~\)` Believe the 5% estimate is more likely to stem from error -- `\(~~\)` We found **no significant effects** on -- <svg viewBox="0 0 512 512" style="position:relative;display:inline-block;top:.1em;fill:grey;height:1.5em;" xmlns="http://www.w3.org/2000/svg"> <path d="M256 8C119.043 8 8 119.083 8 256c0 136.997 111.043 248 248 248s248-111.003 248-248C504 119.083 392.957 8 256 8zm0 448c-110.532 0-200-89.431-200-200 0-110.495 89.472-200 200-200 110.491 0 200 89.471 200 200 0 110.53-89.431 200-200 200zm107.244-255.2c0 67.052-72.421 68.084-72.421 92.863V300c0 6.627-5.373 12-12 12h-45.647c-6.627 0-12-5.373-12-12v-8.659c0-35.745 27.1-50.034 47.579-61.516 17.561-9.845 28.324-16.541 28.324-29.579 0-17.246-21.999-28.693-39.784-28.693-23.189 0-33.894 10.977-48.942 29.969-4.057 5.12-11.46 6.071-16.666 2.124l-27.824-21.098c-5.107-3.872-6.251-11.066-2.644-16.363C184.846 131.491 214.94 112 261.794 112c49.071 0 101.45 38.304 101.45 88.8zM298 368c0 23.159-18.841 42-42 42s-42-18.841-42-42 18.841-42 42-42 42 18.841 42 42z"></path></svg> `\(~~~\)` Credibility of the results -- <svg viewBox="0 0 512 512" style="position:relative;display:inline-block;top:.1em;fill:grey;height:1.5em;" xmlns="http://www.w3.org/2000/svg"> <path d="M256 8C119.043 8 8 119.083 8 256c0 136.997 111.043 248 248 248s248-111.003 248-248C504 119.083 392.957 8 256 8zm0 448c-110.532 0-200-89.431-200-200 0-110.495 89.472-200 200-200 110.491 0 200 89.471 200 200 0 110.53-89.431 200-200 200zm107.244-255.2c0 67.052-72.421 68.084-72.421 92.863V300c0 6.627-5.373 12-12 12h-45.647c-6.627 0-12-5.373-12-12v-8.659c0-35.745 27.1-50.034 47.579-61.516 17.561-9.845 28.324-16.541 28.324-29.579 0-17.246-21.999-28.693-39.784-28.693-23.189 0-33.894 10.977-48.942 29.969-4.057 5.12-11.46 6.071-16.666 2.124l-27.824-21.098c-5.107-3.872-6.251-11.066-2.644-16.363C184.846 131.491 214.94 112 261.794 112c49.071 0 101.45 38.304 101.45 88.8zM298 368c0 23.159-18.841 42-42 42s-42-18.841-42-42 18.841-42 42-42 42 18.841 42 42z"></path></svg> `\(~~~\)` Confidence in the aggregate parameter estimate of 5% -- <svg viewBox="0 0 512 512" style="position:relative;display:inline-block;top:.1em;fill:grey;height:1.5em;" xmlns="http://www.w3.org/2000/svg"> <path d="M256 8C119.043 8 8 119.083 8 256c0 136.997 111.043 248 248 248s248-111.003 248-248C504 119.083 392.957 8 256 8zm0 448c-110.532 0-200-89.431-200-200 0-110.495 89.472-200 200-200 110.491 0 200 89.471 200 200 0 110.53-89.431 200-200 200zm107.244-255.2c0 67.052-72.421 68.084-72.421 92.863V300c0 6.627-5.373 12-12 12h-45.647c-6.627 0-12-5.373-12-12v-8.659c0-35.745 27.1-50.034 47.579-61.516 17.561-9.845 28.324-16.541 28.324-29.579 0-17.246-21.999-28.693-39.784-28.693-23.189 0-33.894 10.977-48.942 29.969-4.057 5.12-11.46 6.071-16.666 2.124l-27.824-21.098c-5.107-3.872-6.251-11.066-2.644-16.363C184.846 131.491 214.94 112 261.794 112c49.071 0 101.45 38.304 101.45 88.8zM298 368c0 23.159-18.841 42-42 42s-42-18.841-42-42 18.841-42 42-42 42 18.841 42 42z"></path></svg> `\(~~~\)` Perceived impact of bias on the 5% estimate -- `\(~~\)` For the additional **exploratory measure**, lay consumers of multi-analyst studies with **consistent** results <svg viewBox="0 0 512 512" style="position:relative;display:inline-block;top:.1em;fill:green;height:1.5em;" xmlns="http://www.w3.org/2000/svg"> <path d="M8 256C8 119 119 8 256 8s248 111 248 248-111 248-248 248S8 393 8 256zm143.6 28.9l72.4-75.5V392c0 13.3 10.7 24 24 24h16c13.3 0 24-10.7 24-24V209.4l72.4 75.5c9.3 9.7 24.8 9.9 34.3.4l10.9-11c9.4-9.4 9.4-24.6 0-33.9L273 107.7c-9.4-9.4-24.6-9.4-33.9 0L106.3 240.4c-9.4 9.4-9.4 24.6 0 33.9l10.9 11c9.6 9.5 25.1 9.3 34.4-.4z"></path></svg> `\(~~~\)` Believe the 5% estimate is more likely to stem from idiosyncratic choices ??? Contrary to our hypotheses, we find that lay consumers of multi-analyst studies with consistent results also have lower beliefs and believe the 5% estimate is more likely to stem from error or from idiosyncratic choices. We found no significant effects on the credibility of the results, confidence in the aggregate parameter estimate of 5%, and the perceived impact of bias on the estimate. --- background-image: url("talk/Figure2.jpg") background-position: 50% 50% background-size: 78% ??? Note. Prior beliefs are displayed in blue; posterior beliefs are displayed in orange. The respective boxes display the lower quartiles, medians, and upper quartiles of prior and posterior beliefs by condition. It is worth noting on the basis of Figure 2 and a post-hoc, paired t-test that, while multi-analyst studies with consistent results perform worse or no better than single-analyst studies on all measures, there is a significant, positive effect of the findings on posterior beliefs within the multi-consistent condition: i.e., beliefs in the research hypothesis are greater after reading the multi-consistent study results, M_d=4.75, p<.001. This finding clarifies that multi-analyst studies are not necessarily bad in absolute terms — however, when comparing to conventional, single-analyst scientific research, crowdsourced data analysis does not seem to provide an improvement in the sway and credibility of scientific research to lay consumers. --- class: inverse, center, middle name: discussion # Discussion --- `\(~~\)` .center[ ### **Compared to a single estimate, we find no evidence that crowd estimates improve lay perceptions of scientific findings** ] `\(~~\)` ??? We conclude that, compared to a single estimate, we do not find evidence that crowd estimates improve lay perceptions of scientific findings. In the future, we are curious to explore whether it might be aversion to variability that explains these findings, and what the perceptions of scientists are. We also believe that in the future it is important for crowd scientists to consider how -- for example, by drawing from research on science communication -- to tackle science skepticism and effectively communicate variable crowd estimates to lay consumers. From the proliferation of big team science and large-scale replication initiatives to preregistration and registered reports, several scientific fields have undergone significant reform with the well-intended goal of improving the reliability of scientific research. The multi-analyst approach has many worthy uses, from demonstrating the arbitrariness and impact of individual analytic choices to acknowledging the inherent variability of results and averaging across idiosyncratic analytic choices to obtain more accurate parameter estimates. However, as with any real-world intervention, scientific reform can have unintended consequences. Here, we focus on the effects of crowdsourcing data analysis, and find that the multi-analyst approach may have an unintended consequence. While partly instituted with the goal of improving the credibility of scientific research, lay consumers appear to resist the inherent variability and lack of consensus that often reflect the reality of multi-analyst research. To our surprise, even when results generated by independent analysts are largely consistent, lay consumers are less likely to believe in the reported phenomenon and more likely to think that the findings stem from error or idiosyncratic choices. -- ## Future directions <svg viewBox="0 0 512 512" style="position:relative;display:inline-block;top:.1em;fill:#035AA6;height:1.5em;" xmlns="http://www.w3.org/2000/svg"> <path d="M256 8C119.043 8 8 119.083 8 256c0 136.997 111.043 248 248 248s248-111.003 248-248C504 119.083 392.957 8 256 8zm0 448c-110.532 0-200-89.431-200-200 0-110.495 89.472-200 200-200 110.491 0 200 89.471 200 200 0 110.53-89.431 200-200 200zm107.244-255.2c0 67.052-72.421 68.084-72.421 92.863V300c0 6.627-5.373 12-12 12h-45.647c-6.627 0-12-5.373-12-12v-8.659c0-35.745 27.1-50.034 47.579-61.516 17.561-9.845 28.324-16.541 28.324-29.579 0-17.246-21.999-28.693-39.784-28.693-23.189 0-33.894 10.977-48.942 29.969-4.057 5.12-11.46 6.071-16.666 2.124l-27.824-21.098c-5.107-3.872-6.251-11.066-2.644-16.363C184.846 131.491 214.94 112 261.794 112c49.071 0 101.45 38.304 101.45 88.8zM298 368c0 23.159-18.841 42-42 42s-42-18.841-42-42 18.841-42 42-42 42 18.841 42 42z"></path></svg> `\(~~\)` Does **variability aversion** explain the findings? ??? We conclude that, compared to a single estimate, we do not find evidence that crowd estimates improve lay perceptions of scientific findings. In the future, we are curious to explore whether it might be aversion to variability that explains these findings, and what the perceptions of scientists are. We also believe that in the future it is important for crowd scientists to consider how -- for example, by drawing from research on science communication -- to tackle science skepticism and effectively communicate variable crowd estimates to lay consumers. -- <svg viewBox="0 0 448 512" style="position:relative;display:inline-block;top:.1em;fill:#035AA6;height:1.5em;" xmlns="http://www.w3.org/2000/svg"> <path d="M437.2 403.5L320 215V64h8c13.3 0 24-10.7 24-24V24c0-13.3-10.7-24-24-24H120c-13.3 0-24 10.7-24 24v16c0 13.3 10.7 24 24 24h8v151L10.8 403.5C-18.5 450.6 15.3 512 70.9 512h306.2c55.7 0 89.4-61.5 60.1-108.5zM137.9 320l48.2-77.6c3.7-5.2 5.8-11.6 5.8-18.4V64h64v160c0 6.9 2.2 13.2 5.8 18.4l48.2 77.6h-172z"></path></svg> `\(~~\)` Perceptions of **scientists**? -- <svg viewBox="0 0 576 512" style="position:relative;display:inline-block;top:.1em;fill:#035AA6;height:1.5em;" xmlns="http://www.w3.org/2000/svg"> <path d="M532 386.2c27.5-27.1 44-61.1 44-98.2 0-80-76.5-146.1-176.2-157.9C368.3 72.5 294.3 32 208 32 93.1 32 0 103.6 0 192c0 37 16.5 71 44 98.2-15.3 30.7-37.3 54.5-37.7 54.9-6.3 6.7-8.1 16.5-4.4 25 3.6 8.5 12 14 21.2 14 53.5 0 96.7-20.2 125.2-38.8 9.2 2.1 18.7 3.7 28.4 4.9C208.1 407.6 281.8 448 368 448c20.8 0 40.8-2.4 59.8-6.8C456.3 459.7 499.4 480 553 480c9.2 0 17.5-5.5 21.2-14 3.6-8.5 1.9-18.3-4.4-25-.4-.3-22.5-24.1-37.8-54.8zm-392.8-92.3L122.1 305c-14.1 9.1-28.5 16.3-43.1 21.4 2.7-4.7 5.4-9.7 8-14.8l15.5-31.1L77.7 256C64.2 242.6 48 220.7 48 192c0-60.7 73.3-112 160-112s160 51.3 160 112-73.3 112-160 112c-16.5 0-33-1.9-49-5.6l-19.8-4.5zM498.3 352l-24.7 24.4 15.5 31.1c2.6 5.1 5.3 10.1 8 14.8-14.6-5.1-29-12.3-43.1-21.4l-17.1-11.1-19.9 4.6c-16 3.7-32.5 5.6-49 5.6-54 0-102.2-20.1-131.3-49.7C338 339.5 416 272.9 416 192c0-3.4-.4-6.7-.7-10C479.7 196.5 528 238.8 528 288c0 28.7-16.2 50.6-29.7 64z"></path></svg> `\(~\)` Importance of **science communication** and **communicating uncertainty** -- <svg viewBox="0 0 352 512" style="position:relative;display:inline-block;top:.1em;fill:#035AA6;height:1.5em;" xmlns="http://www.w3.org/2000/svg"> <path d="M176 80c-52.94 0-96 43.06-96 96 0 8.84 7.16 16 16 16s16-7.16 16-16c0-35.3 28.72-64 64-64 8.84 0 16-7.16 16-16s-7.16-16-16-16zM96.06 459.17c0 3.15.93 6.22 2.68 8.84l24.51 36.84c2.97 4.46 7.97 7.14 13.32 7.14h78.85c5.36 0 10.36-2.68 13.32-7.14l24.51-36.84c1.74-2.62 2.67-5.7 2.68-8.84l.05-43.18H96.02l.04 43.18zM176 0C73.72 0 0 82.97 0 176c0 44.37 16.45 84.85 43.56 115.78 16.64 18.99 42.74 58.8 52.42 92.16v.06h48v-.12c-.01-4.77-.72-9.51-2.15-14.07-5.59-17.81-22.82-64.77-62.17-109.67-20.54-23.43-31.52-53.15-31.61-84.14-.2-73.64 59.67-128 127.95-128 70.58 0 128 57.42 128 128 0 30.97-11.24 60.85-31.65 84.14-39.11 44.61-56.42 91.47-62.1 109.46a47.507 47.507 0 0 0-2.22 14.3v.1h48v-.05c9.68-33.37 35.78-73.18 52.42-92.16C335.55 260.85 352 220.37 352 176 352 78.8 273.2 0 176 0z"></path></svg> `\(~~~\)` Other **suggestions**? --- class: inverse, center, middle # Thank you!